In the dynamic landscape of banking and finance, maintaining a strategic edge in technology is essential for sustainable growth and success. Given the rapid growth of artificial intelligence solutions in the banking sector, leading fintech companies are not merely acquainted with the concept of machine learning; they are actively refining and optimizing their AI initiatives.
That’s why in this article, we decided to help you discover all key use cases of Machine Learning in the banking sector, including customer service and fraud detection solutions, process automation tools for risk management, and regulatory compliance. We will dive deep into real-world examples of how leading financial institutions are leveraging ML to transform their operations, optimize their processes, and strengthen customer engagement.
Machine Learning in Banking – Market Overview
Banking and Finance are among the biggest adopters of Machine Learning, with generative Artificial Intelligence in the Banking and Finance Market forecasted to reach USD 12,337.87 million by 2032, according to Precedence Research.
McKinsey estimates throughout the cross-section of Banking, Wholesale, and Retail, generative AI could add between USD 200 billion to USD 340 billion in value for businesses across the globe. From the investment perspective, things are also looking brighter than ever as in 2023, the Financial industry invested an estimated USD 35 billion in AI, with the Banking sector leading the charge, accounting for approximately USD 21 billion, as stated in the report by Statista.
With those impressive numbers in mind, let’s shift to discussing what exact value Machine Learning systems bring to the table.
The Importance of Leveraging ML in Banking and Finance
AI/ML innovations are already changing how financial institutions do business, and more fascinating improvements are yet to come. In this section, we will look at the most impactful benefits that can be already achieved.
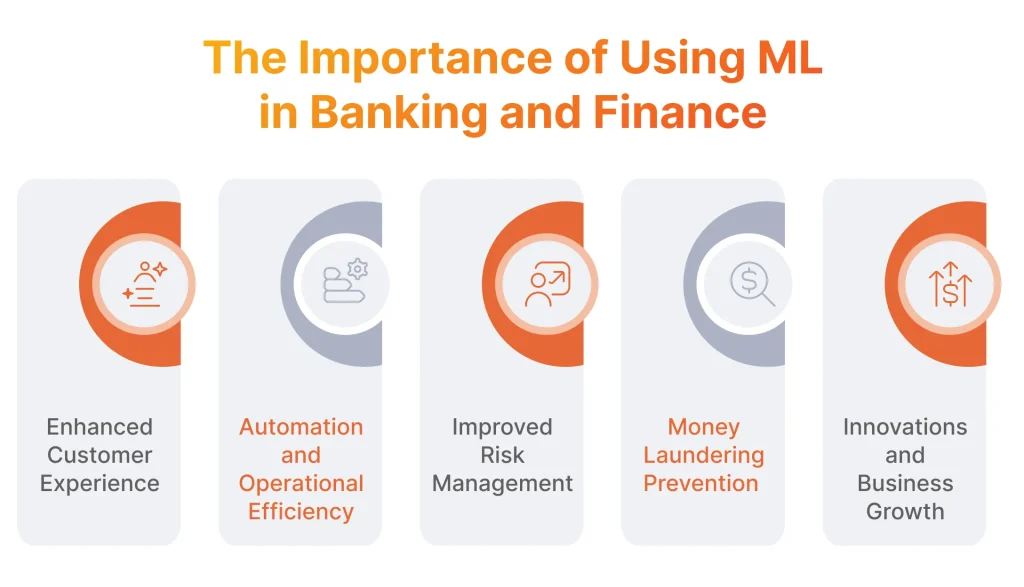
Enhanced Customer Experience
By analyzing customer data, ML algorithms can predict individual preferences, anticipate needs, and offer relevant products and services. The business impact of Big Data combined with Machine Learning, when done right, is truly remarkable and allows banks to deliver personalized and tailored customer experiences.
By the way, the same principles work for Demand Forecasting with Machine Learning, which we described in one of our previous articles, and talked about forecasting methods in more detail.
Below are some of the ways to use AI and ML to meet customer expectations and exceed them.
- Predictive Customer Service: ML enables banks to anticipate customer needs and proactively address their concerns. By analyzing historical data and customer interactions, Machine Learning algorithms can predict potential issues or questions that customers may have and offer timely assistance. Whether it’s resolving bank account inquiries, providing actionable personalized financial advice, or alerting customers about suspicious transactions, AI for customer behavior analysis enhances the overall customer experience by reducing wait times, minimizing frustration, and fostering trust and customer loyalty.
- Fraud Detection and Prevention: Machine Learning also plays a crucial role in enhancing security and fraud prevention measures, safeguarding customers’ financial assets, and maintaining trust. By analyzing transaction data in real time, ML models can detect suspicious activities, identify fraudulent patterns, and flag potentially fraudulent transactions for further investigation.
Credit card fraud detection is one of the most powerful ways to implement Machine Learning in Banking.
Read our article to obtain an in-depth perspective!
Automation and Operational Efficiency
ML automation streamlines manual processes and repetitive tasks, allowing the achievement of reduced operational costs and enhanced efficiency. Tasks such as data entry, document processing, and customer inquiries can be automated using ML algorithms, freeing up human resources for higher-value activities. Process automation can also improve accuracy and reduce errors, leading to faster transaction processing and minimizing operational disruptions, and all of these benefits come from a single document management system.
We know first-hand how automation of data processing can help an organization, as we leveraged ML and OpenAI for automated data collection and processing in B2B intelligence services in one of our cases. The results for our client were truly impressive, as we managed to minimize operational costs 5x due to the fact that our product offers faster task completion, contributing to enhanced staff efficiency.
Improved Risk Management
Algorithms can analyze vast amounts of financial and transaction data to identify patterns, trends, and anomalies, enabling banks to assess and mitigate various types of risks more effectively. ML-driven risk models provide deeper insights and more accurate predictions than traditional approaches and legacy credit risk models. By leveraging predictive analytics and real-time monitoring, banks can proactively identify and respond to emerging risks and safeguard assets.
Money Laundering and Terrorism Financing Prevention
With the power of AI, it is possible to analyze transaction data to detect suspicious activities, identify user spending patterns that seem unusual, and flag potentially fraudulent transactions for further investigation. By leveraging advanced analytics and anomaly detection techniques, banks can enhance their Anti-Money Laundering (AML) and Know Your Customer (KYC) processes, ensuring compliance with regulatory requirements and mitigating the risk of financial crimes.
Innovations and Business Growth
Financial institutions can experience growth by unlocking new opportunities for product development, market expansion, and revenue generation. ML algorithms enable banks to uncover hidden insights from data, identify untapped market segments, and develop innovative products and services tailored to customer needs. Whether it’s launching AI-powered robo-advisors for investment management, introducing chatbots for reliable customer service solutions, or implementing predictive analytics for loan underwriting, ML-driven innovations empower banks to stay ahead of the competition, adapt to changing market dynamics, and capitalize on emerging trends.
Predictive analytics in Retail is a great example of what benefits businesses can reap from AI technology advancements in other massive industries. Banking can easily benefit from such Retail use cases as optimized pricing strategies or tailored marketing campaigns.
Back-Office Use Cases of Machine Learning in Banking
Now let’s focus on specific use cases divided by key categories of operations relevant for financial institutions.
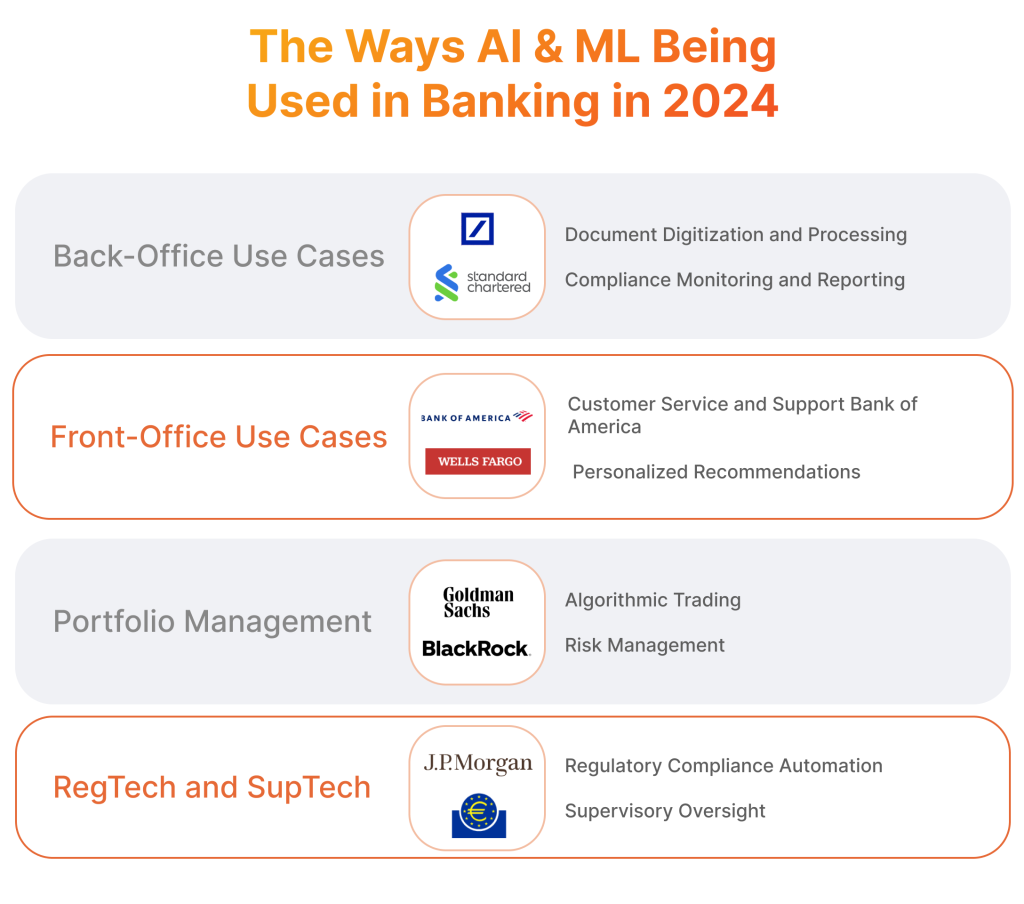
Document Digitization and Processing
Document digitization involves converting physical documents into digital formats, such as PDFs or images, for easier storage, retrieval, and processing. AI-powered Optical Character Recognition (OCR) technology plays a key role in digitizing paper documents by extracting text and data points from scanned images, while Robotic Process Automation (RPA) helps to enter data from various sources.
Document processing, on the other hand, involves analyzing and interpreting digitized documents to extract relevant information, validate data, and perform necessary actions based on the document content. AI algorithms can automate various document processing tasks, such as classification, extraction, validation, and routing.
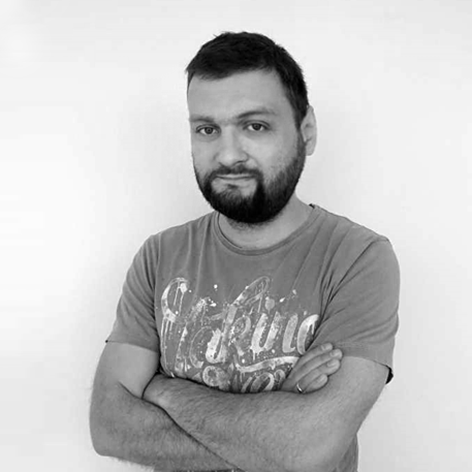
Serhii Leleko
ML & AI Engineer at SPD Technology
“Integrating AI-powered document processing solutions to an existing ecosystem and workflows of your organization requires careful planning and coordination. You need to be ready for additional preparation activities to ensure seamless integration without the disruptions of critical business processes.”
Real World Example
Deutsche Bank has been using AI solutions to automate manual processes and to improve document classification, extraction, and validation tasks in their back-office as well as customer advisory services. The most prominent cases include speeding up the highly manual process of checking and processing loan documents thanks to Optical Character Recognition, using AI to optimize client portfolios in Wealth Management, and monitoring transactions for suspected cases of financial crime.
The company is committed to leveraging the huge potential of AI in a responsible, secure, and prudent manner, making an additional effort to ensure that banking algorithms are fair, don’t include any hidden discrimination, and that results are factually correct and explainable.
Compliance Monitoring and Reporting
Monitoring transactions and analyzing data points to detect suspicious activities is a critical component of compliance with regulatory requirements, particularly in the banking industry where financial institutions are required to prevent money laundering, terrorist financing, and other unlawful activities. Machine Learning algorithms play a crucial role in this process by automating the detection of suspicious patterns, anomalies, and unusual behaviors in transactional data.
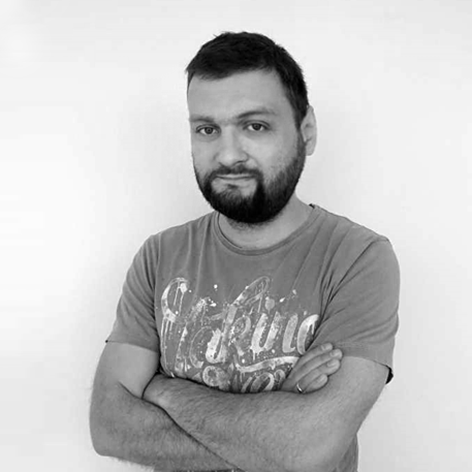
Serhii Leleko
ML & AI Engineer at SPD Technology
“It is crucial to have high-quality data available for accurate detection of suspicious activities. There is also a challenge because ML models may lack interpretability, making it hard to explain the rationale behind alert generation or risk-scoring decisions, so it’s essential to work on constant improvement of the model’s interpretability.”
Real World Example
Standard Chartered Bank utilizes Machine Learning-based transaction monitoring systems to enhance its Anti-Money Laundering (AML) efforts and comply with regulatory requirements. In addition to that, this bank is also using AI and ML to automate many of its manual processes and improve the efficiency of its operations. The Bank is also actively using AI to gain a better understanding and proactively monitor customer behavior for providing a more personalized experience.
Front-Office Use Cases of ML in Banking Services
Now let’s move on to applications that directly impact customer-facing interactions, products, and services of financial institutions. Here are some of the most interesting examples of tools that drive customer loyalty in the banking sector.
Customer Service and Support
EMARKETER forecasts that by the end of 2024, 33.2% of adult consumers in the US will use AI-enabled banking chatbots. There’s no surprise in this remarkable popularity, as chatbots offer several benefits for customers, including:
- 24/7 Availability
- Instant Responses
- Assisting in Transactions
- Continuous learning based on interactions
Real World Example
Bank of America is making waves in the Banking and Finance industry with Erica, an AI-powered virtual assistant, that provides personalized financial guidance and assistance to their customers, enhancing overall service and adding to customer loyalty.
Erica stands out among other banking apps by leveraging the latest technologies, in advanced analytics and cognitive messaging, to serve as a trusted financial assistant. Erica takes into account a range of data within Bank of America, like customer’s cash flow, balances, transaction history, and upcoming bills, to help customers stay on top of their finances.
Personalized Product Recommendations
Machine Learning algorithms analyze customer data, including transaction history, spending patterns, and demographics, to generate personalized product recommendations and tailored offers. Personalized product recommendations are increasingly becoming a cornerstone of the Banking sector, enabling banks to meet the unique needs and preferences of individual customers. As J.D. Power study finds that 78% of banking consumers expect personalized support from their bank.
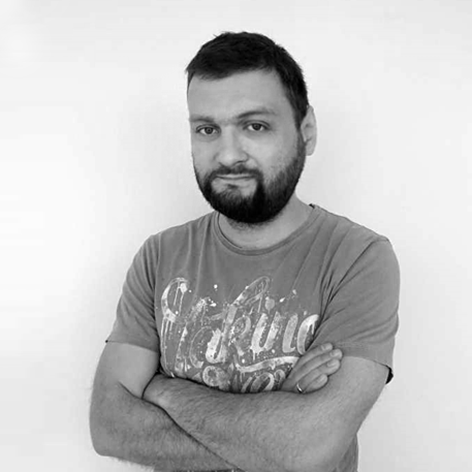
Serhii Leleko
ML & AI Engineer at SPD Technology
“Machine Learning models are not exclusive to delivering outstanding personalized services. Some of the other important AI techniques should be mentioned, as well, including Collaborative Filtering, Content-Based filtering, and Reinforcement Learning.”
Real World Example
Wells Fargo leverages Machine Learning engines for recommendations in their operations. Their solution is called Customer Engagement Engine, and it suggests relevant banking products and services to customers, improving cross-selling opportunities and customer satisfaction. Customer Engagement Engine identifies customer needs and then delivers what they call ‘next best conversations’ in real-time.
Product personalization is also a very significant use case of Machine Learning and Artificial Intelligence in the food industry.
Discover more about its promising applications in our detailed article on this topic!
Trading and Portfolio Management Use Cases
These are fundamental aspects of the Banking and Financial Services sector, and the following Machine Learning solutions play a pivotal role in optimizing these functions.
Algorithmic Trading
Algorithmic trading, powered by Machine Learning (ML) algorithms, has already revolutionized the financial markets by enabling automated trading strategies that can analyze market data, identify trading opportunities, and execute trades with minimal human intervention.
The most common use cases include:
- Market Data Analysis: where ML algorithms analyze vast amounts of market data, including currency changes, news sentiment, and macroeconomic indicators.
- Pattern Recognition: identifying patterns, trends, and correlations in historical market data to predict future price movements and trading opportunities.
- Trading Strategy Optimization: algorithms can help traders here by optimizing trading strategies based on historical performance, market conditions, and risk preferences.
- Execution Automation: ML algorithms can execute trades automatically based on predefined trading rules, signals, and parameters.
- Market Impact Analysis: the market impact of executed trades can be analyzed, and marketing trading strategies can be adjusted accordingly to minimize transaction costs.
- High-Frequency Trading (HFT): ML algorithms enable high-frequency trading strategies that capitalize on short-term market inefficiencies and exploit microstructural patterns in market data.
Real World Example
Goldman Sachs utilizes Machine Learning for algorithmic trading across various asset classes, leveraging AI to make data-driven trading decisions and enhance trading performance.
Their solution is called Goldman Sachs Electronic Trading (GSET) and it offers a comprehensive suite of algorithms to help clients achieve their trading objectives. Their algorithms are designed to leverage both historical analyses and real-time market information, making the strategies dynamic tools to help clients navigate a variety of market conditions.
Risk Management and Portfolio Optimization
Risk management and portfolio optimization are two critical operations within the Banking industry. Machine Learning technologies play a crucial role in enhancing these areas by enabling more sophisticated analysis, modeling, and decision-making processes.
Risk Management use cases for Machine Learning in banking include:
- Credit Risk Modeling: ML algorithms analyze customer data, credit histories, financial statements, and macroeconomic indicators to assess creditworthiness, predict default probabilities, and quantify credit risk exposures.
- Market Risk Management: the analysis of market data, including price movements, volatility, correlations, and macroeconomic indicators, to assess market risk exposures, measure portfolio sensitivities, and manage trading risks.
- Liquidity Risk Monitoring: AI systems monitor liquidity metrics, cash flows, funding sources, and market liquidity conditions to assess liquidity risk exposures, identify liquidity constraints, and manage funding liquidity risks.
Portfolio Optimization solutions may include:
- Asset Allocation Strategies Optimization: delivering asset allocation strategies by analyzing historical data, risk-return tradeoffs, and investor preferences to construct diversified portfolios that maximize risk-adjusted returns.
- Factor-Based Investing: ML algorithms identify and analyze factors that drive asset returns, such as market factors, sector factors, style factors, and macroeconomic factors, to construct factor-based investment asset management strategies and smart beta portfolios.
- Risk Parity Strategies: optimizing risk parity strategies by balancing risk contributions across portfolio assets, ensuring equal risk allocations and diversification benefits across asset classes.
Real World Example
BlackRock is a great example of using Machine Learning to improve risk management and portfolio optimization. Their solution is called Aladdin Risk, and it combines sophisticated risk analytics with quality-controlled data and highly scalable processing capabilities.
BlackRock provides a comprehensive view of portfolio risk and performance, including:
- Portfolio positioning and exposures
- Performance and attribution
- Portfolio risk and scenario analysis
- Asset allocation analysis
- Compliance and oversight
- Security risk and interactive analytics
Aladdin tech produces and monitors 5000+ risk factors daily, with 300+ risk exposures and metrics being reviewed daily.
RegTech and SupTech Use Cases
Regulatory Technology (RegTech) and Supervisory Technology (SupTech) are areas where AI is being increasingly applied to address regulatory compliance challenges and enhance supervisory oversight capabilities.
Regulatory Compliance Automation
The automation of this area involves leveraging AI technologies to streamline and optimize compliance monitoring, reporting, and risk assessment processes:
- The compliance monitoring area includes transaction monitoring, Know Your Customer (KYC) checks, and conducting Customer Due Diligence (CDD).
- As for reporting, regulatory reporting, Suspicious Activity Reporting (SAR) and audit trail generation can be automated.
- Risk assessment can benefit from automated risk modeling, scenario analysis, and compliance risk mapping.
Real World Example
JPMorgan Chase employs AI-powered compliance monitoring systems to detect and prevent fraudulent transactions and ensure compliance with regulatory standards, reducing compliance risks and improving regulatory transparency. It is only one use case in their broad AI Research Program that also includes areas like predicting and affecting economic systems, eradicating financial crime, perfecting client experience, as well as establishing ethical and socially good AI.
Supervisory Oversight and Monitoring
This involves regulatory authorities using various tools and techniques to assess the health of financial institutions and the stability of the financial system as a whole. Machine Learning has become increasingly important in this regard, enabling regulators to analyze large volumes of data, identify emerging risks, and enhance their supervisory capabilities.
The key areas that Machine Learning can contribute to include:
- Analysis of Systemic Risks
- Financial Stability Monitoring
- Identifying Potential Vulnerabilities.
Real World Example
The European Central Bank (ECB) has been developing SupTech Machine Learning tools in collaboration with national supervisors to address several pressing needs. More than a dozen of these have already gone live, and they include:
- Athena, a web-based platform that helps supervisors find, extract, and compare information from millions of articles, supervisory assessments, and bank documents.
- Heimdall, a custom-made tool that streamlines and enhances assessments through automatic Machine Reading powered by Optical Character Recognition to fit proper questionnaires, enables automatic translation, provides textual analysis, and delivers process automation and data analytics capabilities.
- IMAS, the Information Management System, which has grown together with European banking supervision. It is tailor-made to serve the ecosystem of supervisors and supervised entities alike, digitalizing their interactions, enhancing data quality, and increasing transparency and efficiency.
We have another article that focuses solely on Machine Learning in Finance.
Feel free to read it if you want to discover more details on AI and Machine Learning adoption in this related industry!
Machine Learning in Banking Future Trends and Applications
The potential use cases will only get more interesting with the rapid adoption of AI and ML. Here is an overview of the most promising ones at the moment.
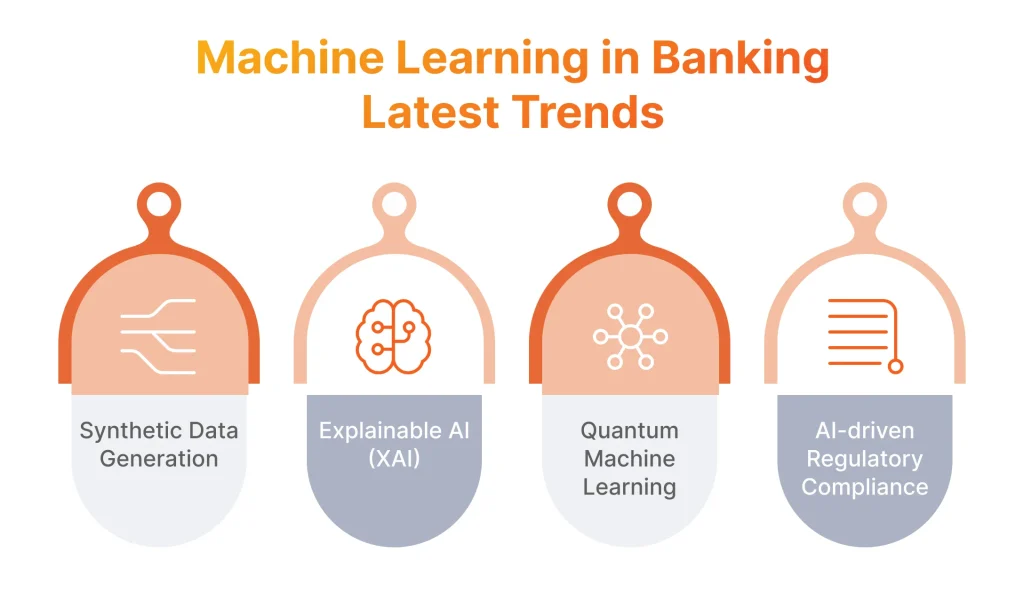
Synthetic Data Generation
Powered by generative AI, Synthetic Data Generation, presents an innovative approach for financial institutions to create realistic yet synthetic data that mirrors real customer data while safeguarding privacy and complying with data protection regulations like GDPR. This synthetic data serves as a valuable resource for training Machine Learning models, refining Fraud Detection algorithms, and enhancing customer analytics without compromising sensitive information.
Here’s a closer look at potential applications of this in the Banking industry:
- Anti-Money Laundering (AML) Compliance: Generative AI enables banks to produce synthetic transaction data that mimics real-world scenarios, allowing them to test and validate their AML detection systems more comprehensively. By generating diverse synthetic data sets representing various transaction patterns and behaviors, banks can enhance the effectiveness and accuracy of their AML algorithms while ensuring compliance with regulatory requirements.
- Fraud Detection and Prevention: Synthetic data generation empowers banks to create simulated fraud scenarios for training and improving their Fraud Detection models. This approach enables banks to stay ahead of evolving fraud tactics while safeguarding customer assets and maintaining trust in the financial system. A somewhat similar concept is widely adopted for Artificial Intelligence and Machine Learning in manufacturing to achieve improved Quality Control by creating diverse examples of normal and abnormal conditions.
- Credit Risk Assessment: Banks can leverage generative AI to generate synthetic credit data, including demographic information, credit scores, and historical financial behavior, for assessing credit risk and predicting loan defaults. Synthetic data allows banks to augment their existing data sets, particularly in scenarios where data scarcity or privacy concerns limit the availability of real customer data. By generating synthetic data that closely resembles real credit profiles, banks can enhance the accuracy and reliability of their credit risk assessment models, enabling more informed lending decisions.
- Risk Management and Stress Testing: Generative AI enables banks to simulate various economic scenarios and generate synthetic financial data for stress testing their risk management models. By creating synthetic data sets representing different market conditions, economic shocks, and regulatory changes, banks can evaluate the resilience of their portfolios and assess their exposure to potential risks.
Fraud detection using Machine Learning models has proven to be a very effective approach for financial institutions.
We welcome you to read our article for more details!
Explainable AI (XAI)
This is a critical area of research and development that focuses on making the processes and decisions of AI models more transparent and understandable to humans. By employing various techniques and methods, XAI aims to enhance the interpretability of AI models, enabling users to comprehend how these models arrive at their predictions or decisions in great detail.
Here are some ways in which XAI can be used in the banking and finance sector:
- Feature Importance Analysis: XAI techniques can assist banks in understanding which factors contribute most significantly to credit risk predictions. For instance, when assessing loan applications, banks rely on AI models to evaluate factors such as income, debt-to-income ratio, credit history, and more. XAI methods, such as feature importance analysis, provide insights into the relative importance of these factors in determining creditworthiness.
- Interpretable Trading Models: In algorithmic trading, AI-driven models analyze vast amounts of market data to make trading decisions. However, the rationale behind these decisions may not always be clear to traders and stakeholders. XAI techniques can enhance the interpretability of trading models by providing insights into the factors influencing trading decisions, such as trend analysis, pattern recognition, or sentiment analysis.
- Personalized Recommendations: Banks are already leveraging AI to deliver personalized product recommendations based on customer financial behavior, transaction history, and preferences. However, customers may be skeptical or hesitant to accept these recommendations without understanding why exactly they were chosen. XAI enables banks to explain the underlying factors and reasoning behind personalized recommendations, fostering trust and confidence among customers.
- Adversarial Attack Detection: AI-based fraud detection systems are susceptible to adversarial attacks, where malicious actors attempt to deceive the model by manipulating input data. XAI techniques can help banks detect and mitigate such attacks by identifying patterns of malicious behavior or attempts to disrupt the model. By making AI systems more transparent and interpretable, XAI enables banks to check model predictions and detect anomalies or inconsistencies indicative of adversarial attacks.
Quantum Machine Learning
Quantum Machine Learning (QML) is a cutting-edge interdisciplinary field that merges the principles of quantum computing with Machine Learning algorithms to tackle complex computational problems more efficiently than classical methods. QML harnesses the unique properties of quantum systems, such as superposition, entanglement, and interference, to perform computations on large datasets and enhance the optimization of Machine Learning models.
Here’s how QML can revolutionize the Banking industry:
- Portfolio Optimization: Traditional portfolio optimization involves dealing with numerous investment variables and constraints, which can become computationally intensive. QML algorithms, however, have the capability to handle these complexities more effectively, providing banks with more accurate and robust portfolio optimization solutions. By leveraging QML, banks can make data-driven investment decisions that lead to improved portfolio performance and risk management.
- Quantum Encryption and Security: Quantum encryption techniques leverage the principles of quantum mechanics to establish secure communication channels that are resistant to hacking. These encryption methods rely on the unique properties of quantum systems, such as Quantum Key Distribution (QKD), to ensure the confidentiality and integrity of banking transactions and communications.
- Algorithmic Trading: Traditional algorithmic trading models often face challenges in processing large datasets and performing complex calculations in real time. QML algorithms excel in handling these tasks by leveraging the computational power of quantum systems. By harnessing QML algorithms, banks can gain insights into market trends, predict price movements, and execute trading decisions with greater speed and accuracy.
AI-Driven Regulatory Compliance
Regulatory compliance can benefit greatly from AI-powered innovations in the following ways:
- Regulatory Monitoring and Alerting: AI systems are already continuously monitoring regulatory changes and updates from various sources such as government websites, regulatory bodies, and industry publications. These systems leverage Natural Language Processing (NLP) techniques to analyze vast amounts of regulatory texts and identify relevant updates or changes. By automating this process, banks can stay abreast of regulatory developments in real time, ensuring timely adjustments to their compliance strategies and policies.
- Compliance Reporting and Documentation: Through Machine Learning algorithms, AI systems can parse disparate data sources, including transaction records, customer information, and financial statements, to compile comprehensive reports required by regulatory authorities. By automating this task, AI streamlines the compliance reporting process, reduces manual effort, and minimizes the risk of inaccuracies or inconsistencies in regulatory filings.
- Transaction Monitoring and Surveillance: AI algorithms monitor transactions in real time to detect anomalies, unusual patterns, or potential compliance violations. These algorithms analyze transactional data against predefined rules and historical patterns to identify suspicious activities such as money laundering, terrorist financing, or insider trading.
- Contract Data Management Services and Due Diligence: The review and analysis of contracts and legal documents can be automated to ensure compliance with regulatory requirements and contractual obligations. By leveraging Natural Language Processing for businesses, banks can extract key clauses, terms, and conditions from contracts, enabling compliance teams to assess regulatory implications and identify potential risks efficiently.
Conclusion
The wider adoption of Machine Learning in the Banking and Financial Services industry represents a paradigm shift in how financial institutions operate and serve their customers. Through advanced algorithms and data analytics techniques, Machine Learning models help banks enhance efficiency, improve risk management, and deliver personalized experiences to customers.
From credit scoring and anomaly detection to customer segmentation and personalized marketing, Machine Learning algorithms empower banks to make data-driven decisions, mitigate risks, and drive business growth more than ever before.
Those financial institutions embracing AI are expected to evolve, surpassing the competition. We, at SPD Technology, are here to help you maximize the efficiency of your Machine Learning systems and take your business on the path of digital transformation with intelligent solutions in place!
FAQ
- What Are the Most Common Machine Learning Problems in the Banking Sector and How to Solve Them?
In the Banking industry, some of the most common Machine Learning solutions include:
- Assessing historical consumer loan data to determine the creditworthiness of loan applicants based on their financial history, demographics, and other relevant factors.
- Identifying fraudulent transactions or activities by analyzing patterns and anomalies in financial transaction data.
- Dividing customers into segments based on their behavior, preferences, and other characteristics to tailor marketing strategies and services.
- Predicting which customers are likely to leave the bank or switch to another institution, allows proactive retention efforts.
- Analyzing and predicting various types of risks, such as market risk, operational risk, and credit risk, to optimize risk mitigation strategies.
- What is One Application of Machine Learning in Retail Banking and Finance?
One of the most prominent applications of Machine Learning and Artificial Intelligence for Retail, Banking sector, and Finance is fraud detection. Machine Learning algorithms can analyze vast amounts of transaction data in real time to identify unusual patterns or behaviors that deviate from typical customer activity. By recognizing anomalies, such as unusually large transactions, transactions from unfamiliar locations, or irregular spending patterns, banks can flag potentially fraudulent activities for further investigation or block them outright to prevent financial losses.