The manufacturing industry has undergone digital transformation, driven by advancements in Big Data analytics, artificial intelligence, machine learning, and robotics. McKinsey’s research underscores the tangible benefits, including reductions in machine downtime by 30% to 50% and decreases in quality-related costs by 10% to 20%, among other advantages.
Without a doubt, AI and ML are game-changers for production processes just like they are for operations in many other industries. So, let’s delve into how machine learning in manufacturing enhances operations, explore real-life examples, and glimpse into the future of this advanced technology.
How Machine Learning Improves Manufacturing
The impact of machine learning is felt at every stage of manufacturing. But how exactly does ML help? Let’s explore this in detail.
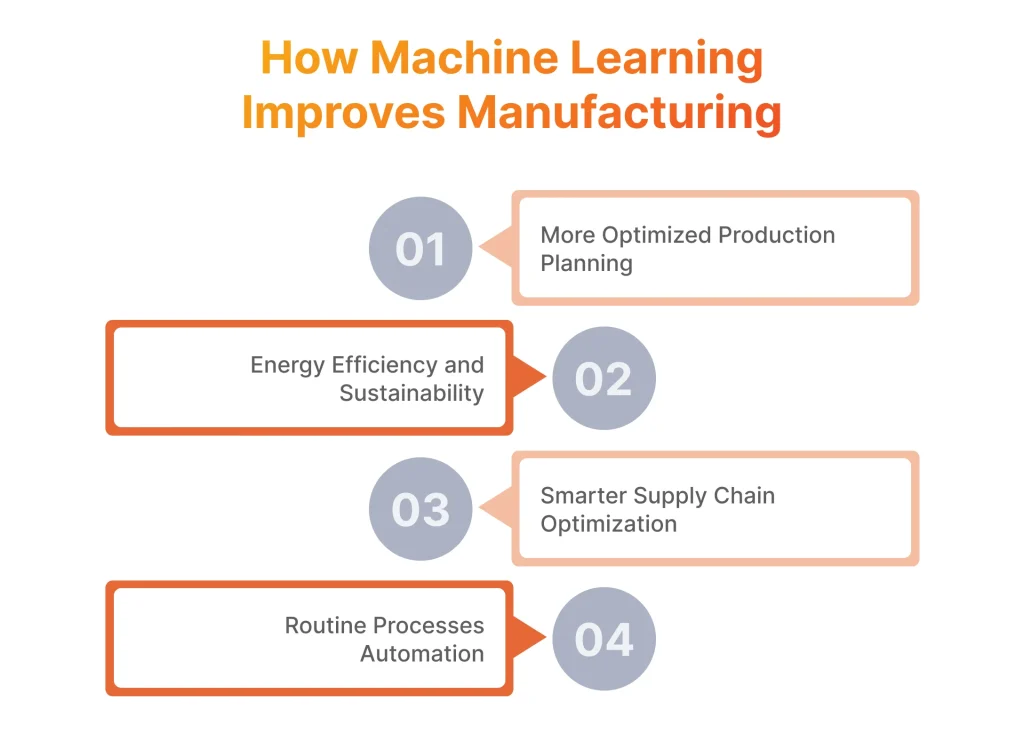
More Optimized Production Planning
Machine learning can assist a manufacturing facility to elevate production processes and achieve greater productivity. Multiple machine learning algorithms can be used to collect and analyze data on production schedules, inventory levels, machine performance, supply chain logistics, and market demand. Powered with that data, machine learning models enable demand forecasting, optimize inventory levels, allow for resource allocation, and streamline production processes.
Moreover, with the help of advanced ML learning algorithms and IoT integration, manufacturers can achieve predictive maintenance and forecast equipment failures by analyzing data from sensors and maintenance logs. These sensors collect different performance indicators (e.g. temperature or pressure), which are then sent to a centralized system. ML algorithms process this data, identifying patterns and anomalies that may indicate malfunctions, and provide manufacturers with insights on when it is time to schedule maintenance.
Want to know more about demand forecasting with machine learning?
Find more information in our featured article!
Energy Efficiency and Sustainability
To fight high levels of energy consumption in manufacturing, machine learning powers data analysis, providing information on energy consumption during production processes. Neural networks identify opportunities for power usage optimization. For example, it can highlight periods of low production where energy can be scaled back.
Beyond analysis, AI automates smart processes. It can dynamically adjust equipment settings for optimal efficiency and even automate equipment shutdowns during idle periods, eliminating unnecessary energy consumption.
The AI’s impact also reaches the implementation of renewable energy sources for production. For that, machine learning modeling helps manufacturing companies to evaluate the environmental impact of manufacturing activities to lower greenhouse gas emissions and carbon footprint.
Smarter Supply Chain Optimization
ML-powered supply chain management opens multiple ways to effectively meet production demands and adapt to dynamic market conditions. With the help of machine learning algorithms manufacturers can obtain highly accurate demand forecasts to optimize inventory levels, preventing stockouts or overstocking. Additionally, AI streamlines supplier selection and management, identifying reliable partners by analyzing quality, reliability, and cost.
As for another use case of AI within supply chain optimization, intelligent algorithms analyze vast amounts of data to plan the most efficient delivery routes. This leads to optimized logistics and distribution channels, which in turn reduces transportation costs. Moreover, AI/ML technologies help to establish warehouse control, allowing for effective layout design and storage assignment.
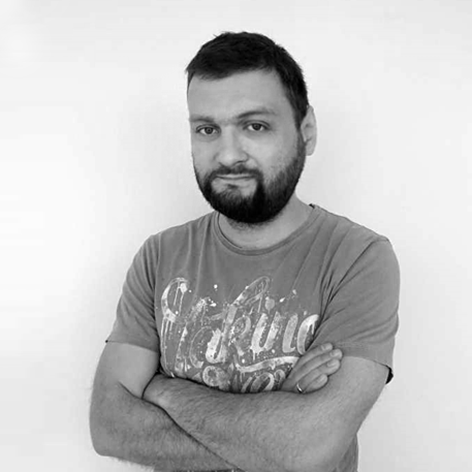
Serhii Leleko
AI & ML Engineer at SPD Technology
“The power of ML-powered supply chains lies in its versatility. We’re not just dealing with static data anymore. By employing diverse algorithms – from time series forecasting for accurate demand prediction to clustering algorithms for optimized warehouse layouts – we can create a truly dynamic system. We are also employing advanced analytics to identify the most reliable suppliers and set dynamic prices that perfectly match market demand.”
Routine Processes Automation
The manufacturing industry can no longer rely on manually performed operations. Therefore, manufacturing companies implement ML-driven automation of material handling and transportation, assembly, packaging and labeling using robots and algorithms. Besides, neural networks analyze input data from various sensors and feedback mechanisms to dynamically adjust assembly tasks, while anomaly detection algorithms automatically identify defects for accurate and time-efficient product inspection.
Machine learning also speeds up data collection and analysis using natural language processing. It helps extract and analyze data from different external systems and process it much faster, reducing human error. For example, at SPD Technology, we helped a packaging manufacturer automate their invoice processing for over 450 vendors. We achieved this by using NLP to automatically collect and understand data from invoices. This automation transformed invoice processing from a weeks-long manual task to one that can now be completed in just hours.
What Advantages AI/ML Brings to Manufacturing Business
With machine learning algorithms leveraged to optimize multiple processes in the manufacturing industry, businesses can unlock a range of benefits. Below we describe some of them.
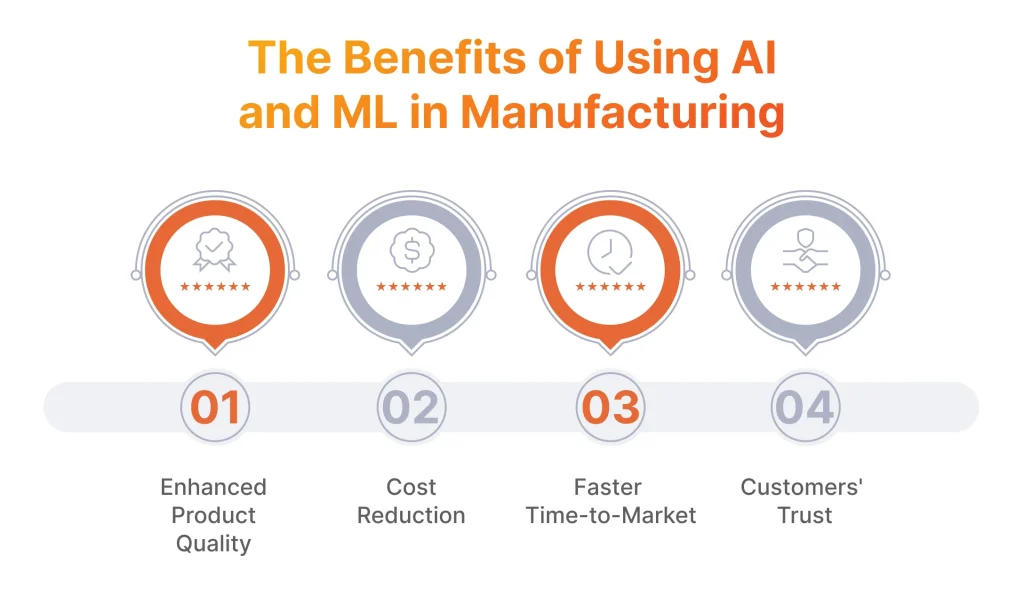
Enhanced Product Quality
Machine learning technologies are revolutionizing manufacturing by bringing precision and eliminating defects. One of the most important concepts for achieving product quality with ML is digital twins. According to the McKinsey survey, 86% of responders agreed that digital twins are applicable in production operations. The essence of the technology, in turn, is that ML-enabled digital twins enable virtual representation of products, reproducing their designs and characteristics for evaluation and testing.
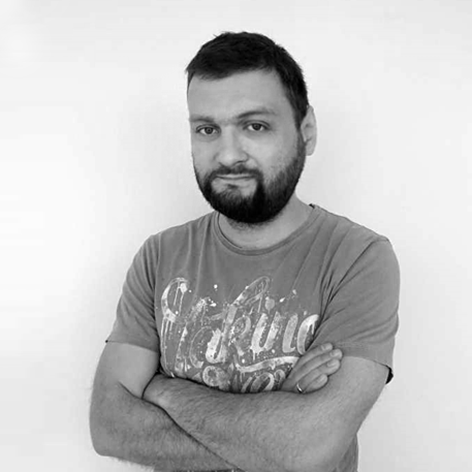
Serhii Leleko
AI&ML Engineer at SPD Technology
“Digital twins are powered by machine learning algorithms. They enable the creation of virtual replicas of products, reproducing their designs and characteristics for evaluation and testing. This technology analyzes data to simulate how the product would perform under different conditions, helping businesses identify design and functionality flaws.”
Cost Reduction
As we mentioned above, artificial intelligence and machine learning in manufacturing contribute to automation of production processes, supply chain management, and repetitive tasks. Hence, all the operations that previously required human efforts can now be performed faster and without human error, significantly reducing the cost.
Machine learning also plays a crucial role in minimizing cost for manufacturing processes by enabling early defect detection. ML-powered visual inspection systems surpass human inspectors in both precision and speed. This allows manufacturers to catch defects earlier in the production line and take the corresponding actions to fix the identified issue.
Another AI-enabled opportunity that leads to cost savings in manufacturing is predictive maintenance with Machine Learning. While traditional maintenance relies on fixed schedules, ML grants manufacturers the ability to forecast potential failures, prevent breakdowns beforehand, and establish uninterrupted production.
Faster Time-to-Market
With automated tasks and predictive maintenance, it’s obvious that manufacturing processes become faster, resulting in timely product launches.
On top of that, manufacturing companies can significantly improve supply chains and optimize logistics with machine learning. Engineers can fine-tune machine learning models to analyze transportation routes, supplier locations, and real-time traffic conditions. For example, one of our tasks for the HaulHub project involved leveraging artificial intelligence to analyze traffic patterns to gain insights into how construction activities impact road traffic before, during, and after construction. In the similar manner, our ML engineers and data scientists can craft machine learning solutions for logistics routes, drastically reducing time required to deliver products to the market.
Customer Trust
The manufacturing sector can benefit from utilizing machine learning models when it comes to sentiment analysis and predicting customer preferences. This is where companies can benefit from the business impact of Big Data and leverage predictive analytics. Its algorithms can forecast customer response to potential product features or designs. This allows manufacturers to focus on concepts that are more likely to resonate with their target audience, streamlining the design process and reducing development time.
Moreover, through sentiment analysis techniques, ML models analyze massive datasets and identify the emotional tone of customer communications from social media posts, online reviews, and customer surveys. By identifying positive, negative, or neutral sentiments, businesses can understand how customers feel about their products.
Want to learn how AI predicts what customers want?
Discover more in our detailed article.
Advanced Use Cases of AI/ML in Manufacturing with Examples
Businesses are rapidly embracing artificial intelligence and machine learning in manufacturing to drive digital transformation and maintain competitiveness. According to Precedence Research, the global market value for AI in manufacturing reached USD 3.8 billion in 2022 and is projected to reach approximately USD 68.36 billion by 2032, with a compound annual growth rate of 33.5% from 2023 to 2032.
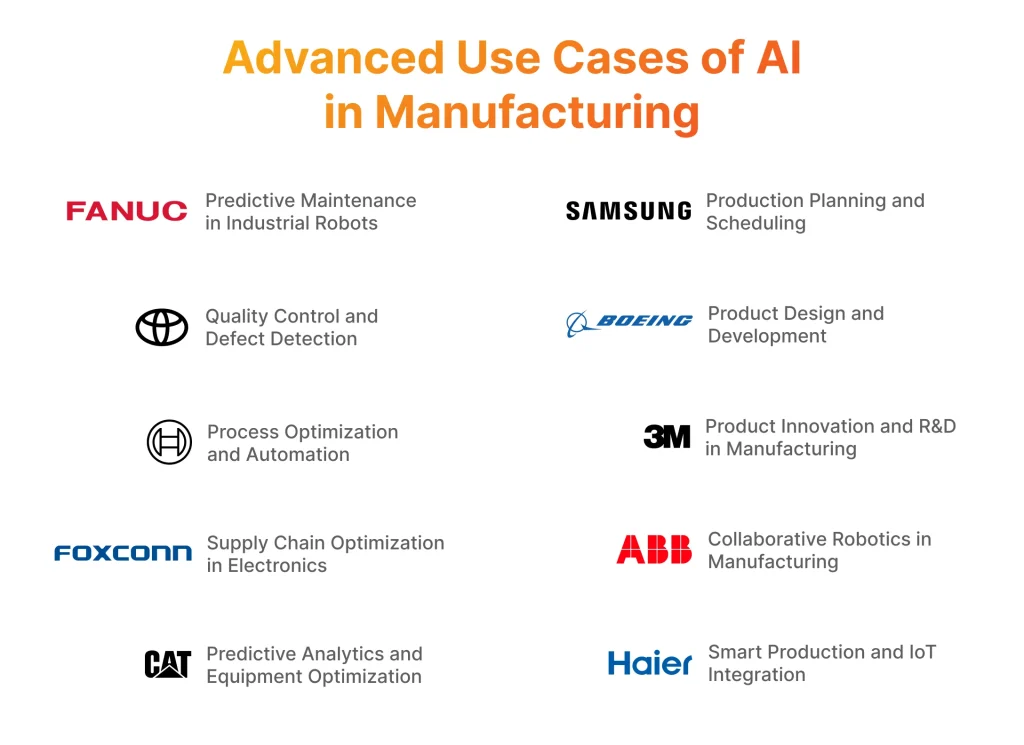
Leading manufacturers are leveraging machine learning technologies across different functions, including planning optimization, supply chain management, and product design. Let’s delve into notable examples of AI/ML utilization by manufacturing industry leaders.
Fanuc Corporation: Predictive Maintenance in Industrial Robots
This Japanese manufacturer of robotics and computer numerical control wireless systems has implemented predictive maintenance for their industrial machines. This decision enables the company to:
- Extend machine lifespan by predicting breakages and allowing for early repairs;
- Improve safety by addressing issues like overheating or malfunctioning components;
- Optimize maintenance cost thanks to targeted repairs and replacements rather than blanket overhauls.
Toyota: Quality Control and Defect Detection in the Automotive Industry
At Toyota Motor Corporation, ML-powered computer vision helps to inspect vehicle components and eliminate defects before cars are launched on the market. Thanks to the integration of AI/ML technology, the leading automotive manufacturer achieves:
- Enhanced accuracy by automating inspection and reducing human errors;
- Faster defect detection through real-time product image analysis;
- Improved product quality thanks to early and consistent defect detection;
- Reduced production costs with automated quality control to minimize fixes and rework.
Bosch: Process Optimization and Automation in Manufacturing
In Bosch manufacturing, machine learning and artificial intelligence bring automation for assembly lines and machining operations. This allows:
- Faster production since repetitive tasks like welding, painting, and parts assembly are free from manual execution;
- Minimized waste as ML-powered automation reduces rebuild;
- Optimized labor costs as human workers are assigned with higher-value tasks.
Foxconn: Supply Chain Optimization in Electronics Manufacturing
A Taiwanese electronics manufacturer Foxconn optimizes inventory management and logistics, ensuring timely delivery of high-quality electronic products globally. Thanks to ML, the company receives the following benefits:
- Lower inventory costs through better forecasting and demand planning;
- Improved supply chain flexibility by effectively adjusting production schedules from alternative suppliers;
- Enhanced collaboration thanks to ML-powered real-time tools that provide instant insights into inventory levels, supplier performance, and potential disruptions.
Caterpillar: Predictive Analytics and Equipment Optimization in Heavy Machinery Manufacturing
Caterpillar leverages AI algorithms to evaluate equipment sensor data, predict equipment failures, and optimize its performance. With predictive analytics tightly integrated with heavy machinery processes, the company ensures:
- Extended equipment lifespan thanks to identifying and addressing equipment failures early;
- Proactive risk mitigation as predictive analytics signals potential safety hazards, such as overheating or pressure fluctuations;
- Continuous improvement since predictive analytics provides production teams with valuable insights into machine performance.
Samsung Electronics: Production Planning and Scheduling in Electronics Manufacturing
Samsung enhances production schedules and resource allocation with AI-powered algorithms. This approach ensures timely delivery of products to meet market demands thanks to:
- Reduced lead times, minimizing delays and bottlenecks throughout the production process;
- Optimized production flow with well-defined schedules and a smooth flow of materials through different stages of assembly;
- Improved labor utilization through effective scheduling that ensures sufficient workforce is available to meet production demands.
Boeing: Product Design and Development in Aerospace Manufacturing
Boeing is advancing aerospace manufacturing through AI-driven digital twins. Using AI and ML, the company gains the following advantages:
- Data-driven maintenance, upgrades and enhancements;
- Improved design iteration thanks to data analysis from simulations and identifying areas for improvement;
- Increased quality control leading to reduced risk of defective components being incorporated into aircrafts.
3M: Product Innovation and R&D in Manufacturing
3M, the company that operates in the field of worker safety, healthcare, and consumer goods, uses AI to lead its R&D efforts. Their data scientists utilize ML algorithms to:
- Collect scientific research data to optimize designs and functionality of existing products;
- Analyze data from various fields to lead innovative solution development.
ABB Robotics: Collaborative Robotics in Manufacturing
ABB Robotics facilitate safe human-robot interaction, allowing robots and workers to collaborate on assembly tasks. As a result of this ML initiative, ABB ensures:
- Reduced human error since robots perform repetitive tasks with more precision;
- Cost savings due to improved operational efficiency.
Haier Group: Smart Production and IoT Integration in Home Appliances Manufacturing
Haier Group’s production teams employ AI and ML to develop smart and connected appliances with improved:
- Process optimization as IoT sensors embedded in machines and production lines can collect real-time data on temperature, pressure, and energy consumption;
- Quality control through ML-powered inspection for early detection of minor defects;
- Demand forecasting by analyzing historical sales data and consumer trends to predict future demand for specific appliance types.
The Future of AI in Production
Artificial intelligence’s growth in manufacturing is changing the rules within the industry. From unprecedented quality control to collaborative robotics, AI’s impact is just beginning. Let’s explore how AI will transform production in the recent future.
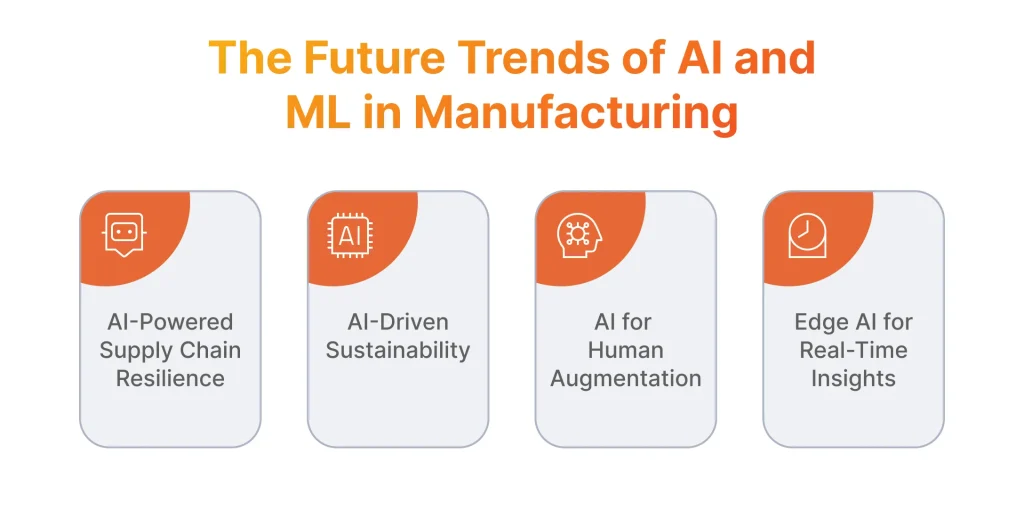
AI-Powered Supply Chain Resilience
Pandemics, geopolitical events, and natural disasters require manufacturers, suppliers, distributors, and retailers to quickly adapt to changing market conditions and ensure uninterrupted services for customer satisfaction. This is why the AI in the supply chain market is projected to reach USD 10,110.2 million by 2025 from USD 730.6 million in 2018.
AI will enhance demand prediction, route optimization, dynamic inventory management and predictive maintenance to transform supply management from reactive to proactive and establish agility, resilience and customer-centric approach to logistics. Moreover, AI-powered supply chains will be integrated with computer vision to enable cameras and sensors to analyze activity in production environments, warehouses, or transportation routes. This will translate into boosted efficiency, accuracy, and safety in the supply chain.
AI-Driven Sustainability
AI drives eco-friendliness of manufacturing businesses by optimizing energy consumption, integrating with technologies for renewable energy, and reducing material usage during production. Moreover, this approach contributes to saving costs, as statistics show that in 2023, all sustainable AI cost-saving measures were reported to have at least a 40 percent impact on enterprise expenses.
Sustainable AI benefits various industries, with the food industry being a prime example. It can help farmers grow more food with fewer resources, reduce environmental impact, and minimize food waste throughout the supply chain.
What to learn about the impact of artificial intelligence on the food industry?
Discover all the details in our article!
Edge AI for Real-Time Insights
Edge AI is a new wave of artificial intelligence that brings the processing power to the device itself, rather than relying solely on the cloud. It empowers the devices at the “edge” of the network like smartphones or smart appliances to process data locally using on-board processors or specialized AI chips.
Edge AI is ideal for factories, facilitating real-time decision-making on production lines and enhancing the security of sensitive manufacturing data, all without needing a constant internet connection. Compared to traditional methods, which often rely on cloud computing and continuous internet connectivity, Edge AI offers faster responses and reduced latency, leading to more efficient and secure operations.
AI for Human Augmentation
The human augmentation market is on track to drastic growth, expected to surge from USD 330.38 billion in 2023 to a staggering USD 1,249.43 billion by 2033. That’s a projected increase of USD 1,249.43 billion, reflecting a significant shift towards human enhancement technologies. AI-powered tools and interfaces will increasingly augment human capabilities, not replace them. Manufacturers will be able equip their factories with AI assistants for safety guidance or embrace human-AI cooperation for enhanced productivity and precision.
Conclusion
The integration of AI and ML in manufacturing powers the industry with optimized production planning, enhanced energy efficiency, smarter supply chain management, and automation of routine tasks. These technologies are not only improving operational efficiency and product quality but also reducing costs and time-to-market. Real-life applications by industry leaders like Toyota, Bosch, Samsung, and Boeing demonstrate the diverse and impactful use of AI and ML.
Looking ahead, AI’s role in manufacturing is set to expand further, driving supply chain resilience, sustainability, real-time insights, and human augmentation. The ongoing digital transformation, fueled by AI and ML, promises to maintain the industry’s competitiveness.
FAQ
- What Is Machine Learning in Manufacturing?
Machine learning in manufacturing analyzes massive amounts of data from sensors, machines, and past production runs. By finding patterns and predicting issues, ML helps manufacturers optimize processes, minimize defects, and ensure consistent quality control. It makes it possible to fine-tune machine settings, identify potential problems before they happen, and ensure consistent quality throughout production.
- How Is AI Used in Manufacturing?
AI, which encompasses machine learning, acts as a game-changer in manufacturing. While AI itself is a broad term, in manufacturing it often refers to the practical applications of ML. These applications leverage data to make predictions and improve processes. This contributes to proactive maintenance, preventing costly downtime and ensuring smooth production. AI is also used for optimizing processes, like analyzing data to identify areas for waste reduction or pinpoint bottlenecks in production lines.
- How Machine Learning Works in Production?
In production, ML devours data (sensor readings, settings) to find patterns. These patterns help predict issues and optimize processes by fine-tuning machines, reducing waste, and preventing defects. The more data it sees, the better it gets at its job.