Nowadays, credit card fraud poses a significant threat to businesses and consumers. According to the Federal Trade Commission (FTC), there were 114,348 reported cases of credit card fraud in 2023. Despite efforts made by credit card companies and financial organizations to prevent fraud, the high number of these incidents compel businesses to seek advanced technological solutions for their customers protection.
While traditional detection systems often struggle to keep pace, credit card fraud detection using machine learning is emerging as the new standard. Let’s delve into why this shift is occurring, from understanding the causes and consequences of credit card abuse to exploring the transformative potential of ML in redefining detection methodologies.
How Does Credit Card Fraud Happen?
Credit card fraud has a multitude of forms, showcasing a diverse array of deceptive tactics.Below, we highlight eight of the most prevalent types.
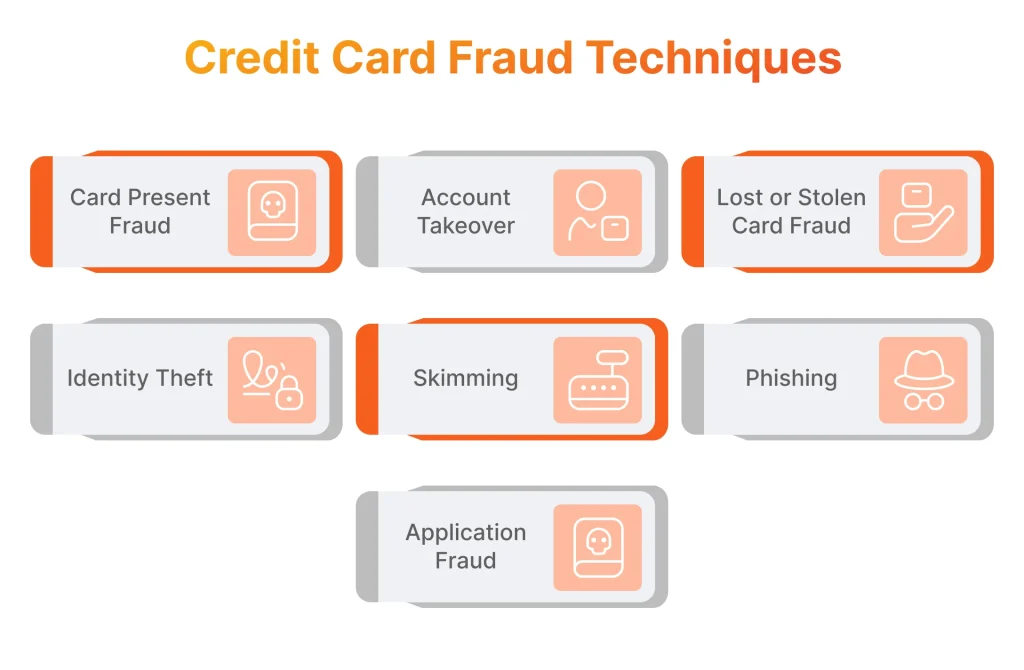
- Card Present Fraud: This type of fraud occurs when a fraudster physically presents a counterfeit or stolen credit card for payment at a merchant location. It may involve using altered or cloned cards to make purchases.
- Card Not Present (CNP) Fraud: CNP fraud happens when the cardholder’s information (such as card number, expiration date, and security code) is stolen and used to make fraudulent transactions online, over the phone, or via mail order. Since the card is not physically present, it’s often easier for fraudsters to carry out these unauthorized transactions.
- Account Takeover: In an account takeover, fraudsters gain unauthorized access to a legitimate cardholder’s account information, often through phishing scams, malware, or data breaches. Once they have control of the account, they make fraudulent purchases, change account details, or transfer funds without the cardholder’s knowledge.
- Lost or Stolen Card Fraud: This fraud occurs when credit cards are physically lost or stolen, and unlawful transactions are made using the cards before the cardholders report them missing. Fraudsters use the stolen card to make purchases or withdraw cash until the card is canceled or blocked.
- Identity Theft: This type of scam involves fraudsters using stolen credit card details, including social security numbers, addresses, and dates of birth, to open new credit card accounts or take over existing ones. They apply for credit cards in the victim’s name and use them for unauthorized transactions, leaving the victim liable for the charges.
- Skimming: In this case, installed devices, or card skimmers, are used on legitimate card readers, such as ATMs, gas pumps, or point-of-sale terminals, to capture card data when the card is swiped or inserted. The stolen credit card details are then used to create counterfeit cards or make unlawful transactions.
- Phishing: These scams involve fraudsters sending deceptive emails, text messages, or phone calls posing as legitimate institutions to trick individuals into revealing their credit card information, login credentials, or other sensitive data. Once obtained, cardholders’ details are used for different types of fraud, including CNP fraud and identity theft.
- Application Fraud: Fraudsters submit fraudulent credit card applications using stolen or fabricated personal information. They use fake identities or stolen documents to obtain credit cards, which are used for unauthorized transactions or sold on the black market.
The Impact of Credit Card Fraud In Facts and Numbers
Fraud cases can have far-reaching consequences not only for individuals but also for organizations. Victims can face financial losses, reputational harm, legal expenses, and disruptions in operations due to fraud. Let’s examine these consequences with examples more closely.
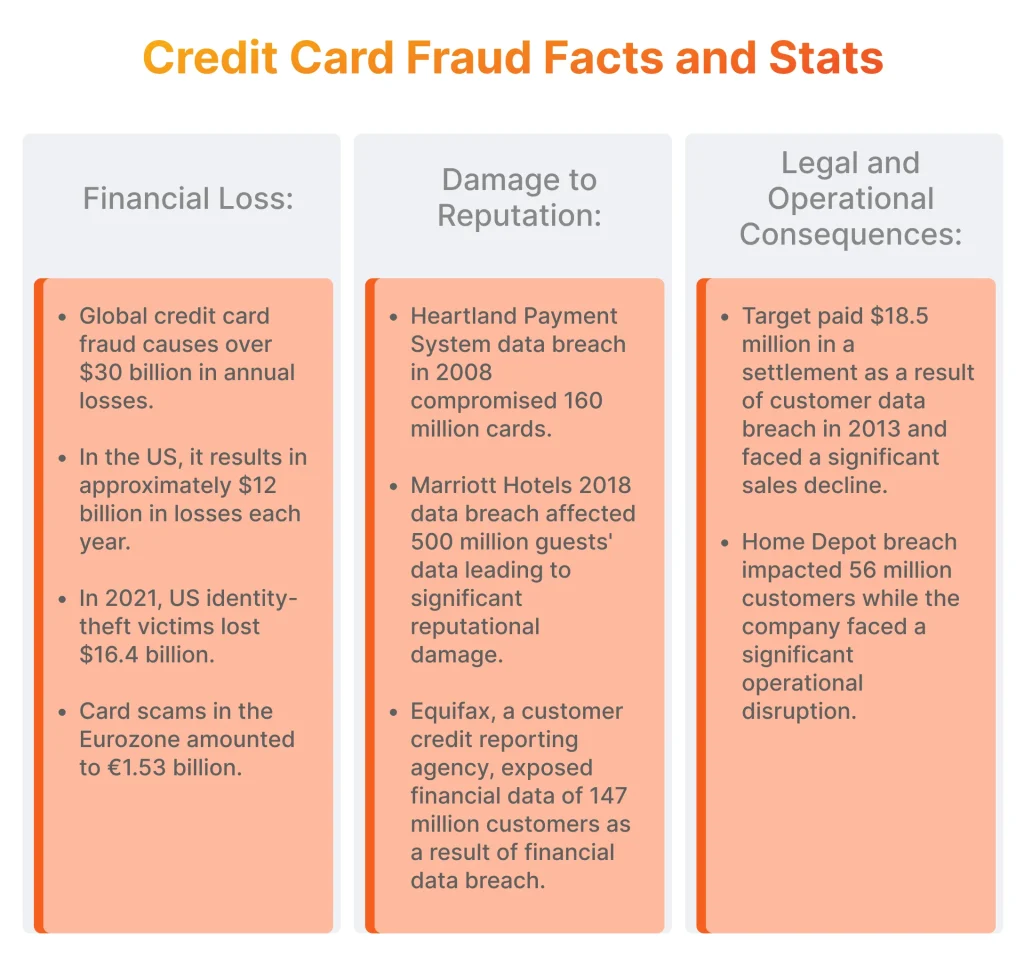
Financial Loss
When fraud occurs, businesses can suffer direct financial losses from fraudulent credit card transactions. They may face additional costs such as chargeback fees, fines, and increased transaction processing expenses associated with implementing fraud prevention measures.
- Credit card fraud results in billions of dollars in losses annually. Globally, merchants and card acquirers have suffered losses exceeding 30 billion U.S. dollars, with approximately 12 billion U.S. dollars stemming from the United States alone.
- In 2021, approximately 59% of identity-theft victims experienced financial losses of $1 or more, amounting to a total of $16.4 billion in the US, as stated by the Bureau of Justice Statistics.
- According to the European Central Bank, card scams constituted 0.028% of the total value of card payments made using cards issued in the Single Euro Payments Area, amounting to €1.53 billion from a total value of €5.40 trillion.
Damage to Reputation
Incidents of financial card fraud damage a business’s reputation and erode consumer trust. Customers start to perceive the business as insecure or unreliable if their transaction data is compromised, leading to decreased sales, negative reviews, and loss of customer loyalty.
- The 2008 data breach at Heartland Payment Systems, caused by malware on its payment processing network, compromised 160 million credit and debit cards. This led to significant financial and reputational damage for the company. Heartland faced numerous lawsuits from financial institutions and cardholders seeking damages for the unauthorized charges and losses incurred as a result of the breach. Even though the data breach occurred in 2008, such reputational consequences remain relevant in 2024.
- The Marriott data breach in 2018 severely damaged the company’s reputation. Hackers gained unauthorized access to the guest reservation database, compromising the personal information, including credit cards, of approximately 500 million guests. Marriott faced significant backlash and negative publicity, with many customers expressing dissatisfaction and concerns about the safety of their personal information.
Legal and Regulatory Consequences
Businesses that fail to implement adequate security measures to protect against fraudulent charges face legal consequences. Depending on the jurisdiction and industry, they are subject to fines, lawsuits, or sanctions for non-compliance with data security standards and consumer protection laws.
- A major retailer Target faced significant legal consequences as a result of the credit card scam incident. The company agreed to pay $18.5 million to 47 states and the District of Columbia in a settlement with state attorneys general. This settlement concluded a years-long investigation into the breach, which compromised the data of millions of customers in 2013. Target also incurred substantial legal fees and costs totaling $202 million.
- Another example is the Equifax data breach in 2017, where hackers gained access to financial data of approximately 147 million consumers. Equifax faced intense legal and regulatory scrutiny, including multiple lawsuits and investigations by state and federal authorities. The company ultimately reached settlements with regulators and agreed to pay significant fines to resolve the legal and regulatory actions against it.
Operational Disruption
Dealing with the aftermath of credit card scam, including investigating incidents, reimbursing affected customers, and implementing fraud prevention measures, can disrupt normal business operations and divert resources away from core activities.
- During the Home Depot data breach in 2014, hackers gained access to Home Depot’s payment systems, compromising the credit and debit card information of approximately 56 million customers. The company experienced a decline in sales and customer trust following the breach, as many customers were concerned about the security of their payment information.
- Operational disruptions were also consequential for Target, mentioned above. The retail chain had to allocate resources to investigate the breach, enhance cybersecurity measures, and provide support to affected customers. Additionally, the breach led to a decline in sales.
Traditional Credit Card Fraud Detection vs Machine Learning
Traditional credit card fraud detection relies on predefined rules and patterns but struggles to keep pace with evolving tactics. In contrast, fraud detection with machine learning harnesses the power of advanced algorithms for identifying patterns of suspicious activity efficiently. The following comparison explores the principles behind both methods in combating fraudulent transactions.
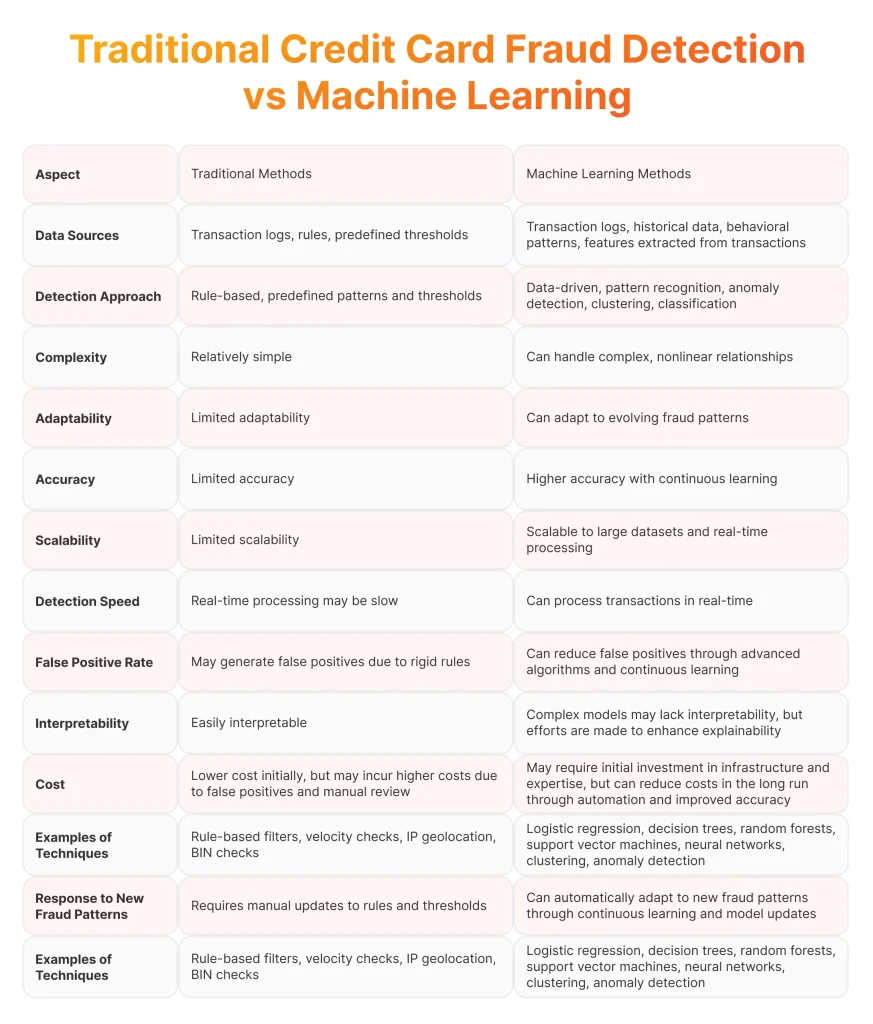
How Machine Learning Helps with Credit Card Fraud Detection?
Compared to traditional fraud detection methods, machine learning revolutionizes card scam detection by offering an array of advantages. Thanks to the following aspects, ML-driven fraud monitoring systems provide unparalleled protection against fraudulent activities.
Improved Accuracy
Machine learning algorithms harness the advantages of big data to discern intricate patterns and anomalies, resulting in unparalleled accuracy in detecting fraudulent activities. By analyzing vast amounts of transaction data and user behavior, ML models can distinguish between legitimate and fraudulent payments with high precision, minimizing false positives and negatives.
Adaptability to New Threats
Continuously learning from fresh data, ML models swiftly identify and counter emerging fraud schemes. Whether it’s discerning sophisticated phishing attempts, combating account takeover fraud, or unveiling novel identity theft techniques, ML systems stay steps ahead by dynamically updating their detection strategies.
Detection of Complex Fraud Schemes
Traditional rule-based fraud detection systems often struggle to detect complex fraud schemes that involve multiple variables and subtle patterns. ML, on the other hand, excels at uncovering hidden correlations and non-linear relationships within data, making it particularly effective in identifying complex fraud schemes. By leveraging advanced algorithms such as neural networks, decision trees, and ensemble methods, ML models can detect even the most sophisticated fraud attempts that may evade traditional detection methods.
Reduced Manual Intervention
ML automates many aspects of the fraud detection process, reducing the need for manual intervention and human oversight. With automation and predictive analytics, ML systems can analyze and flag potentially scam transactions in real-time, allowing fraud analysts to focus their attention on investigating high-risk cases and developing proactive strategies to mitigate fraud.
When Implementing Credit Card Fraud Detection with ML Makes Sense?
In navigating the terrain of credit card fraud detection, certain scenarios emerge where the integration of ML proves particularly advantageous.
High Volume of Transactions
In environments characterized by a high volume of transactions, machine learning can efficiently analyze vast amounts of data in real-time. With its real-time processing capabilities, ML swiftly dissects vast data streams, pinpointing fraudulent patterns amidst the legitimate transactions’ torrential flow.
ML proves extremely advantageous in retail, where there are a substantial number of transactions. ML systems are adept at quickly processing and analyzing extensive datasets, which include transaction specifics, customer interactions, and other pertinent data.
New Market Entry
When entering new markets or expanding globally, businesses may encounter unfamiliar fraud patterns and risks. ML excels in adapting to new environments, leveraging historical data and continuous learning to detect fraudulent behavior unique to specific regions or markets. This adaptability makes ML-based fraud detection particularly suitable for organizations venturing into uncharted territories.
High Risk and Cross Border Transactions
Transactions involving high-risk activities or cross-border transactions are inherently more susceptible to fraud. ML algorithms, with their ability to analyze diverse data sources and detect subtle patterns, provide enhanced capabilities for identifying fraudulent transactions in these scenarios. By meticulously analyzing transaction intricacies, user behavior nuances, and geographical details, ML algorithms meticulously flag suspicious transactions.
Upcoming Integrations with Third-Parties
Expanding business operations through integrations with payment gateways or third-party platforms offer more opportunities but also escalates fraud risks. ML-powered fraud detection can vigilantly monitor transactions within partner networks, swiftly identifying and thwarting fraudulent activities originating from integrated systems.
Discover how to seamlessly integrate a payment gateway into your website, ensuring its security!
The Technical Perspective of Credit Card Fraud Detection with ML
Based on the table above we can say that ML is an advanced method used in finance for card fraud monitoring, preferred by businesses seeking to enhance software security. According to the Statista report, nearly 40% of companies used automation systems, including ML-based solutions, for fraud detection in 2023. But what are the steps involved in preventing fraud using ML? It’s achieved through the following four stages.
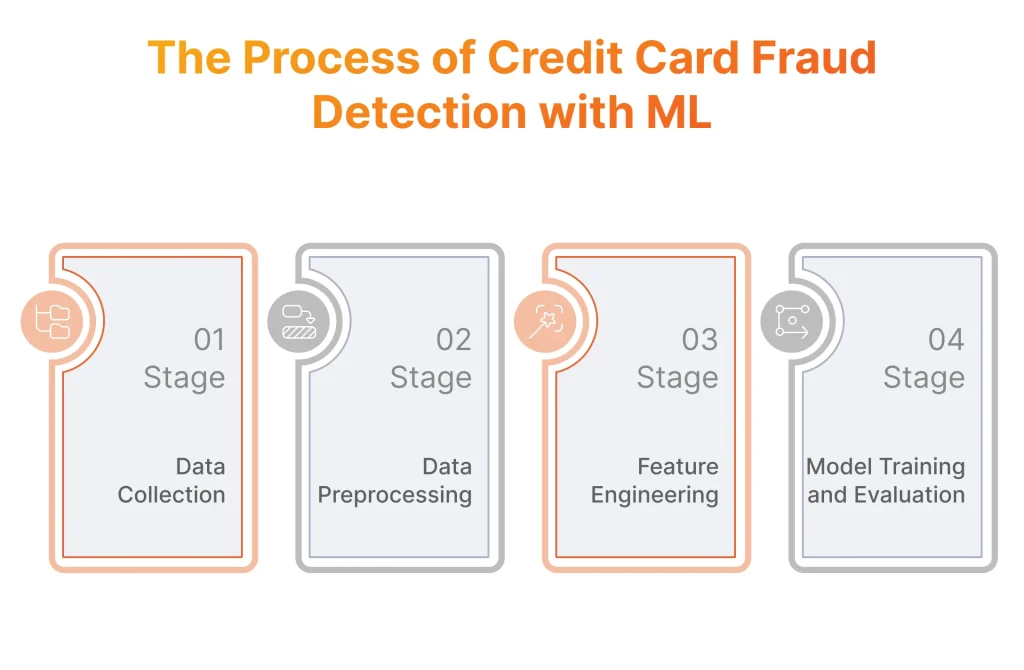
Stage 1: Data Collection
In the context of preventing credit card scam, the first stage involves collecting data from different sources such as transaction logs, customer information databases, device details, external data sources, and historical fraud data. However, the raw data obtained from these sources often contains inconsistencies, missing values, outliers, and other issues that need to be addressed before it can be effectively used to spot frauds.
Stage 2: Data Preprocessing
To clean, transform, and prepare the raw data for analysis, ML engineers apply data preprocessing techniques. This involves several steps:
- Data Cleaning: Involves handling missing values, correcting inconsistencies, and removing duplicates. For example, missing values in transaction amounts or timestamps might be imputed using statistical methods or filled with appropriate defaults.
- Outlier Detection and Handling: Outliers can significantly affect the performance of ML models. Techniques such as Z-score, interquartile range (IQR), or clustering-based approaches can be used to detect and handle outliers.
- Feature Scaling: Features in the dataset may have different scales, which can lead to biased models. Techniques such as normalization or standardization are applied to bring all features to a similar scale.
- Encoding Categorical Variables: Categorical variables such as merchant IDs or transaction types need to be encoded into numerical representations before being fed into ML algorithms. Techniques like one-hot encoding or label encoding are commonly used for this purpose.
- Data Transformation: Transformation techniques like logarithmic transformation or Box-Cox transformation may be applied to make the data more symmetrical and improve model performance.
Stage 3: Feature Engineering
Next comes feature engineering crucial for enhancing the predictive power of ML models in fraud monitoring. It involves creating new features or variables that capture relevant information about fraudulent activities. Feature engineering techniques extract, transform, and aggregate information from the preprocessed data to create new features. Some common feature engineering techniques in monitoring frauds with financial card include:
- Time-Based Features: Features derived from transaction timestamps such as time of day, day of week, or time since last transaction. Fraudulent activities may exhibit specific patterns at short periods of time.
- Transaction Amount Statistics: Aggregations and statistics derived from transaction amounts, such as mean, median, standard deviation, maximum, minimum, and percentiles. Here, anomaly detection with machine learning helps to spot fraudulent behavior.
- Merchant and Customer Behavior Features: Features capturing patterns in merchant-customer interactions, such as frequency of credit card transactions with specific merchants, average transaction amounts per merchant, or unusual changes in spending behavior.
- Device and Location Features: Features related to the device used for transactions (e.g., device ID, IP address) and transaction locations (e.g., geolocation, distance between transaction locations). Sudden changes in device or location may indicate suspicious activity.
- Aggregated Features: Features derived from aggregating historical transaction data, such as the number of previous payments within a certain timeframe, or the frequency of transactions from high-risk locations.
Stage 4: Model Training and Evaluation
After feature engineering, ML models are trained on the preprocessed and feature-engineered data to learn patterns and make predictions. Different ML algorithms such as logistic regression, random forests, gradient boosting, or deep learning models like neural networks are trained on the datasets.
The trained models are evaluated using performance metrics such as, precision, recall, F1-score, and ROC-AUC on a separate test dataset to assess their effectiveness in detecting fraudulent transactions. Techniques like cross-validation and hyperparameter tuning are employed to optimize model performance and prevent overfitting.
Once evaluated, the best-performing model is deployed into production where it continuously monitors incoming payments, flagging potentially suspicious transactions for further review or action.
Machine Learning Models for Fraud Detection
We have already mentioned that ML relies on models to analyze data. These models take features as input and use a set of parameters or weights to process the features and generate an output, which could be a prediction or classification.
Each model has its own architecture and learning algorithms to identify patterns from the features. Let’s examine them more closely based on their learning approaches.
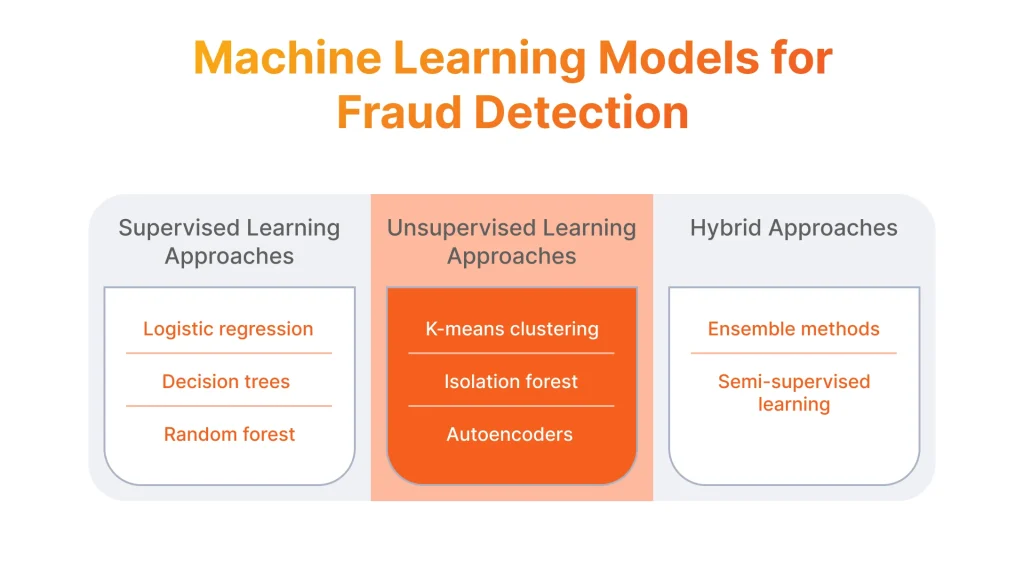
Supervised Learning Approaches
- Logistic regression models the probability that a given instance belongs to a particular class (e.g., fraud or non-fraud) based on its features. It uses the logistic function to transform the output of a linear equation into a probability value between 0 and 1. Except for credit card fraud detection, it is also used for medical diagnosis, and demand forecasting in marketing.
- Decision trees recursively partition the feature space based on feature values to make predictions. At each node of the tree, a decision is made based on the value of a feature, leading to a split in the data. The process continues until a stopping criterion is met, typically when further splits do not improve the purity of the subsets. Decision trees are used in finance, healthcare, and marketing, for tasks such as credit risk assessment, disease diagnosis, and customer behavior analysis for segmentation.
- Random forest builds multiple decision trees on bootstrapped samples of the data and combines their predictions to make a final prediction. Each tree is trained on a random subset of features, reducing the correlation between trees and improving the overall performance and robustness of the model. Besides fraud prevention, random forest is used in spam filtering, and medical diagnosis.
Unsupervised Learning Approaches
- K-means clustering partitions the dataset into k clusters by iteratively assigning data points to the nearest cluster centroid and updating the centroids until convergence. The number of clusters (k) is pre-defined by the user. K-means clustering is commonly used for customer segmentation, image compression, anomaly detection, and predictive analytics in retail for recommendation systems.
- Isolation forest isolates anomalies by randomly selecting a feature and partitioning the data into two subsets. This process is repeated recursively until anomalies are isolated in small partitions. Anomalies, like a fraudulent transaction, are detected as instances that require fewer partitions to separate them from the rest of the data. Isolation forest is used for credit card fraud detection, network intrusion detection, and outlier detection in sensor data.
- Autoencoders consist of an encoder network that compresses the input data into a lower-dimensional representation (encoding) and a decoder network that reconstructs the original input from the encoding. The model is trained to minimize the reconstruction error, forcing it to learn meaningful features from the data. In fraud detection, autoencoders can learn representations of normal behavior and identify deviations as anomalies.
Hybrid Approaches
- Ensemble methods create a diverse set of base models, either by training different models on different subsets of the data (bagging) or by sequentially training models to correct the errors of previous ones (boosting). The predictions of these base models are then aggregated to produce a final prediction. Ensemble methods are widely used for classification, regression, and anomaly detection in multiple industries.
- Semi-supervised learning techniques typically combine supervised learning with unsupervised learning. For example, self-training iteratively trains a model on labeled data and uses it to label unlabeled data, which is then incorporated into the training set for the next iteration. Semi-supervised learning is useful in natural language processing, computer vision, and fraud detection in banking.
Willing to know how ML can transform the banking industry?
Find out in our featured article!
Credit Card Fraud Detection Challenges and Considerations
Using machine learning to detect credit card fraud presents promising opportunities to bolster security and mitigate financial losses. Nevertheless, it also presents several challenges that need to be addressed.
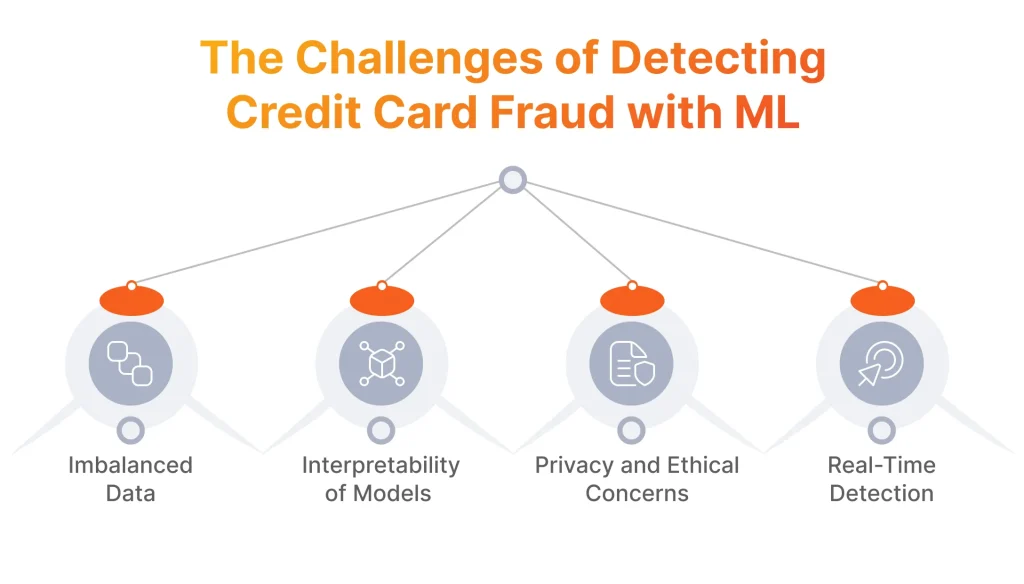
Imbalanced Data
Unlawful transactions are typically rare compared to legitimate ones, resulting in imbalanced datasets where the number of positive (fraudulent) instances is much lower than negative (legitimate) instances. Imbalanced data lead to biased models that prioritize accuracy at the expense of detecting suspicious transactions.
Techniques such as resampling (e.g., oversampling minority class, undersampling majority class), cost-sensitive learning, and ensemble methods help mitigate the imbalance and improve model performance.
Interpretability of Models
The interpretability of fraud detection models is crucial for understanding how they make decisions and gaining insights into the factors contributing to fraudulent behavior. However, complex and opaque models, such as deep learning neural networks, may lack interpretability, making it challenging to trust and explain their predictions.
Employing interpretable models, such as decision trees or logistic regression, whenever possible can enhance transparency and trust in the fraud detection system. Additionally, techniques such as feature importance analysis, model-agnostic interpretability methods, and model documentation help explain the predictions of complex models.
Privacy and Ethical Concerns
Fraud detection systems often process sensitive personal and financial data, raising concerns about privacy violations, data breaches, and ethical considerations. One of the factors that can bring even more risks to privacy is human intervention since it creates the risk of human error or bias.
Implementing robust data protection measures, such as encryption, access controls, and anonymization techniques, is essential for safeguarding customer privacy and complying with data protection regulations. Additionally, automation helps ensure consistent adherence to privacy protocols. It reduces errors and enhances efficiency and scalability in handling large volumes of transactions while maintaining data privacy and ethical standards.
For instance, the Aggregated Merchant Portal (AMP) that we created for Blackhawk Networks, one of our major customers, allows the businesses to automatically pass OFAC (Office of Foreign Assets Control), EIN/SSN (Employer Identification Number / Social Security Number) and other legal entity ID checks.
Real-Time Detection
Detecting fraudulent transactions in real-time is critical to prevent financial losses and minimize the impact on customers. However, traditional ML models may be computationally expensive and time-consuming to process transactions in real-time.
Deploying lightweight and scalable models, leveraging streaming data processing technologies, and implementing efficient feature engineering and model inference pipelines are essential for achieving real-time detection capabilities. Additionally, setting up robust monitoring and alerting systems can help quickly identify and respond to suspicious activities.
Conclusion
Machine learning is a potent weapon against credit card fraud because of its capability to scrutinize vast financial data and identify complex fraudulent patterns. Financial card fraud manifests in different forms, from in-person and online scams to identity theft, necessitating sophisticated detection methods.
The journey of using ML for detecting credit card scam involves several stages, including data collection, preprocessing, feature engineering, model training, and evaluation. Different algorithms and models are employed, each tailored to tackle specific fraud challenges.
Success in spotting fraud with ML depends not just on technical proficiency but also on ethical and privacy considerations. Addressing imbalanced data, interpretability of fraud detection models and real-time detection capabilities also contributes to efficient scam detection.
In essence, ML for card fraud detection signifies a substantial transformation in fortifying transactions across various sectors, including financial institutions and credit card companies. By leveraging data-driven insights and advanced algorithms, businesses and consumers can navigate the digital payment landscape with confidence, knowing they are protected from fraudulent activities.
FAQ
- How Can Machine Learning Detect Credit Card Fraud Transactions?
ML algorithms analyze historical transaction information to identify patterns and anomalies associated with fraudulent activities. Features such as transaction amount, location, time, merchant, and customer behavior are extracted and used to train models. Supervised learning models are trained on labeled data (fraudulent and legitimate transactions) to learn to distinguish between them. Unsupervised learning models detect anomalies by identifying transactions that deviate significantly from normal behavior. Hybrid approaches combine supervised and unsupervised techniques to improve detection accuracy.
- What Are the Algorithms Used for Credit Card Fraud Detection?
The following algorithms, along with various ensemble methods, feature engineering techniques, and model evaluation metrics, are employed in credit card scam detection systems to effectively identify and prevent a fraudulent transaction while minimizing false positives.
- Logistic Regression: Used for binary classification tasks, logistic regression models the probability of a transaction being fraudulent based on its features.
- Decision Trees: Decision trees partition the feature space based on feature values to classify transactions as fraudulent or legitimate.
- Random Forest: An ensemble learning method, it combines multiple decision trees to improve detection accuracy and robustness.
- Neural Networks: Deep learning neural networks, including feedforward, convolutional, and recurrent neural networks, learn complex patterns in financial data for fraud monitoring.
- Isolation Forest: An unsupervised learning algorithm, isolation forest isolates anomalies in transaction information by partitioning the feature space.
- K-means Clustering: Used for grouping transactions into clusters, k-means clustering identifies outliers as potential fraud instances.