Statista believes that by 2030, the global Artificial Intelligence market will reach a staggering USD 1,847,295. This growth is driven by the top industries including Retail, Banking, Manufacturing, and the Healthcare sector is definitely among them.
The adoption rate of Machine Learning in Healthcare is increasing exponentially, with a growing list of use cases from medical imaging analysis to the drug discovery process. According to Grand View Research, the global AI in Healthcare market size was estimated at USD 22.45 billion in 2023 and is expected to expand at a compound annual growth rate (CAGR) of 36.4% from 2024 to 2030. While artificial intelligence is definitely transforming the healthcare landscape, let’s discuss the most prominent ways to apply AI innovations to improve the work of medical professionals in 2024.
How Does Artificial Intelligence Work in Healthcare?
Let’s start with determining what tasks AI helps to accomplish in the Healthcare industry.
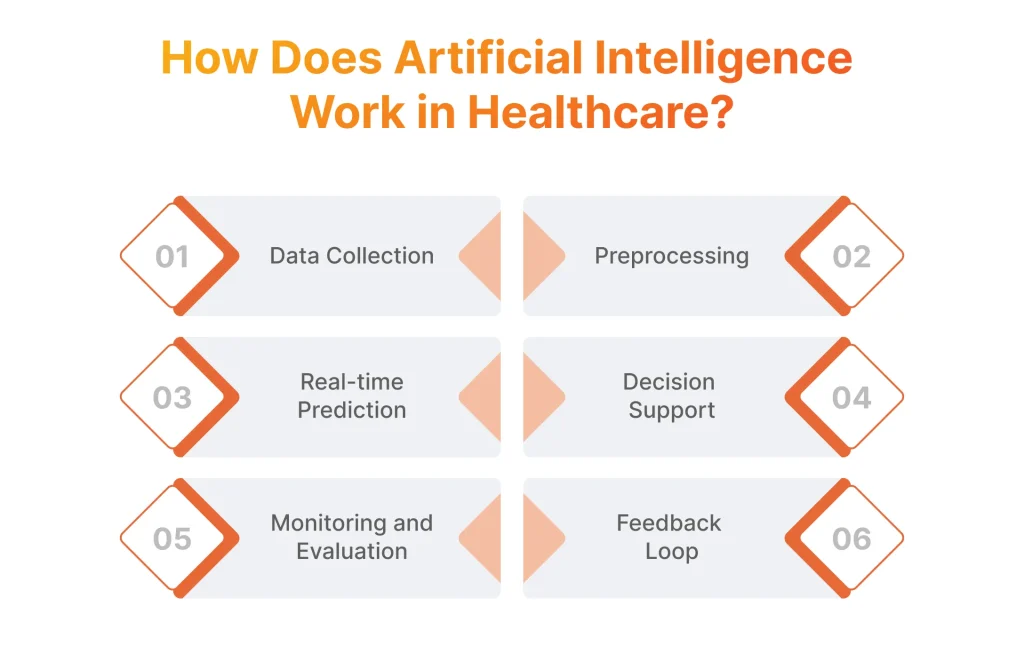
- Data Collection: AI can be a great help in aggregating and managing vast amounts of patient data from diverse sources, including Electronic Health Records (EHRs), wearable devices, and other medical records systems. It is even possible to collect voice data and convert it into text with the help of Natural Language Processing. This comprehensive dataset encompasses critical information such as the patient’s medical history, symptoms, lab results, imaging scans, and treatment outcomes.
- Preprocessing: At the next stage, the trick is that we can’t feed collected data into the Machine Learning algorithms from the get-go since one additional step is required. The preprocessing step is essential to ensure data quality and compatibility. AI algorithms clean noisy data, normalize values, and perform feature engineering to extract relevant information and create meaningful features that enhance the algorithm’s learning process.
- Real-time Prediction: Now, when high-quality data is extracted, Machine learning algorithms can operate in real-time, continuously analyzing incoming patient data to make predictions or classifications. For instance, based on a patient’s symptoms and medical history, AI can predict the likelihood of certain diseases or conditions, enabling timely interventions and personalized care plans. The same concept works perfectly for other industries as well, for example, predictive analytics in Retail, which unlocks the power of Demand Forecasting, Customer Segmentation, and Personalized Recommendations for the industry.
- Decision Support: Automated predictions may serve as invaluable decision-support Machine Learning tools for healthcare providers. By highlighting high-risk patients who require closer monitoring or recommending personalized treatment plans based on individual patient characteristics, AI assists healthcare professionals in making more informed and tailored clinical decisions. Similarly, decision support can be used in other industries, for example, for ML-enabled demand forecasting in Retail.
- Monitoring and Evaluation: Continuous monitoring and evaluation of Machine Learning algorithms are crucial to ensuring their accuracy and effectiveness over time. Healthcare organizations should closely track the algorithm’s performance, identifying any discrepancies or issues promptly to maintain reliability and enhance patient outcomes.
- Feedback Loop: As new data becomes available and additional outcomes are observed, Machine Learning algorithms should undergo periodic updates and retraining to improve their performance and adapt to evolving circumstances. This iterative feedback loop ensures that the algorithms remain responsive to changing patient needs and emerging healthcare trends.
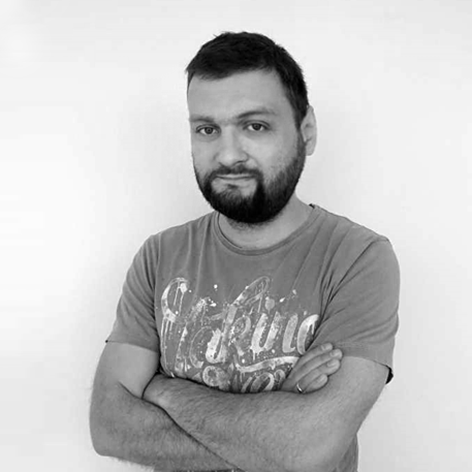
Serhii Leleko
ML & AI Engineer at SPD Technology
“While working with data in the healthcare industry, it is important to always implement robust data governance policies to maintain the accuracy, completeness, and integrity of patient data. Make sure your organization adheres to strict data security and privacy regulations, such as HIPAA, to safeguard patient confidentiality and comply with legal requirements.”
Machine Learning Algorithms Used In Healthcare
It’s time to move on to discussing the most common algorithms that will most likely be used in Machine Learning applications in Healthcare. If you want to discover the basics, we recommend you start with our introduction to Machine Learning.
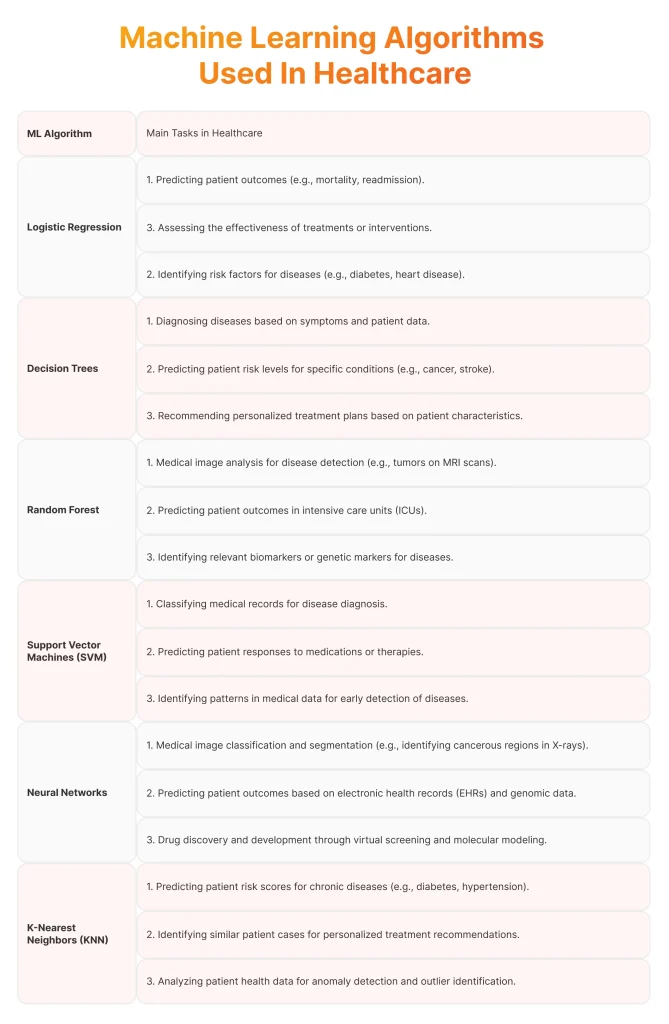
Logistic Regression
This algorithm is known for binary classification tasks, where the outcome is either positive or negative. In the Healthcare industry, it is used to determine the disease diagnosis and the presence or absence of a certain condition. It’s extensively used due to its simplicity, interpretability, and effectiveness in modeling probability. One of the other prominent examples of the usage of this algorithm from other industries includes Fraud Detection with Machine Learning.
The tasks this algorithm helps to accomplish include:
- Predicting the likelihood of developing a disease based on risk factors.
- Distinguishing between patients with and without a specific condition or disease.
- Analyzing risk factors and associations with diseases in epidemiological clinical trials.
Decision Trees
Being popular due to their simplicity and interpretability, they’re suitable for both classification and regression tasks and can handle a mix of numerical and categorical data. Decision trees are often preferred in scenarios where explainability of the model’s decisions is crucial, such as in clinical settings where healthcare professionals and patients need to understand the reasoning behind diagnosis or treatment recommendations.
The tasks these algorithms help to accomplish include:
- Assisting in identifying the most likely diagnosis based on patient symptoms and medical history.
- Suggestion of appropriate treatment options based on patient characteristics.
- Identifying patients at high risk of developing complications or adverse outcomes.
Random Forest
It is an ensemble learning method composed of multiple decision trees that are highly robust and accurate. They excel in handling complex datasets with high dimensionality and noisy or missing data. For healthcare organizations, where datasets are often incomplete, random forest is particularly valuable.
The tasks this algorithm helps to accomplish include:
- Predicting the likelihood of disease onset based on patient characteristics and risk factors.
- Predicting patient outcome, the likelihood of recovery or death.
- Feature importance analysis, identifying the most influential variables or risk factors associated with a particular condition.
Support Vector Machines (SVM)
These are powerful classifiers, particularly effective in handling high-dimensional data and nonlinear relationships. They’re widely used by Healthcare organizations for tasks requiring accurate classification, such as disease diagnosis and patient risk stratification.
The tasks these algorithms help to accomplish include:
- Disease classification, for example, selection between different types or stages of cancer patients, based on medical imaging or genomic data.
- Categorizing patients into risk groups based on clinical variables or biomarkers.
- Medical image analysis, for example, segmenting and classifying tumors in radiological images.
Neural Networks
Neural networks, especially Deep Learning algorithms, and Deep Learning models like CNNs and RNNs, are capable of learning complex patterns and relationships from large volumes of data. They’re highly versatile and find applications across various healthcare domains, from medical image analysis to clinical decision support and genomics.
The tasks this algorithm helps to accomplish include:
- Analysis of medical images, like detecting abnormalities in X-rays, MRIs, or histopathological slides.
- Clinical decision support systems for risk prediction, treatment recommendation, and patient monitoring.
- Analysis of time-series data, such as electronic medical records, to predict patient outcomes and detect anomalies.
K-Nearest Neighbors (KNN)
A simple yet effective algorithm for both classification and regression tasks. It’s particularly useful in scenarios with small to medium-sized datasets and can handle numerical and categorical data points effectively. It is quite popular for anomaly detection with Machine Learning tasks, so we recommend you read our article if you want to learn more.
The tasks this algorithm helps to accomplish include:
- Disease classification tasks, such as predicting the likelihood of a patient having a particular condition based on similar patients in the dataset.
- Patient similarity analysis, identifying individuals with similar medical profiles for personalized treatment plans and recommendations.
- Anomaly detection tasks, identifying outliers in patient vital signs or laboratory results.
The Most Prominent Use Cases of Machine Learning in Healthcare Projects
There are already plenty of interesting ML implementations by healthcare providers. In this section, we will review the ones that truly stand out.
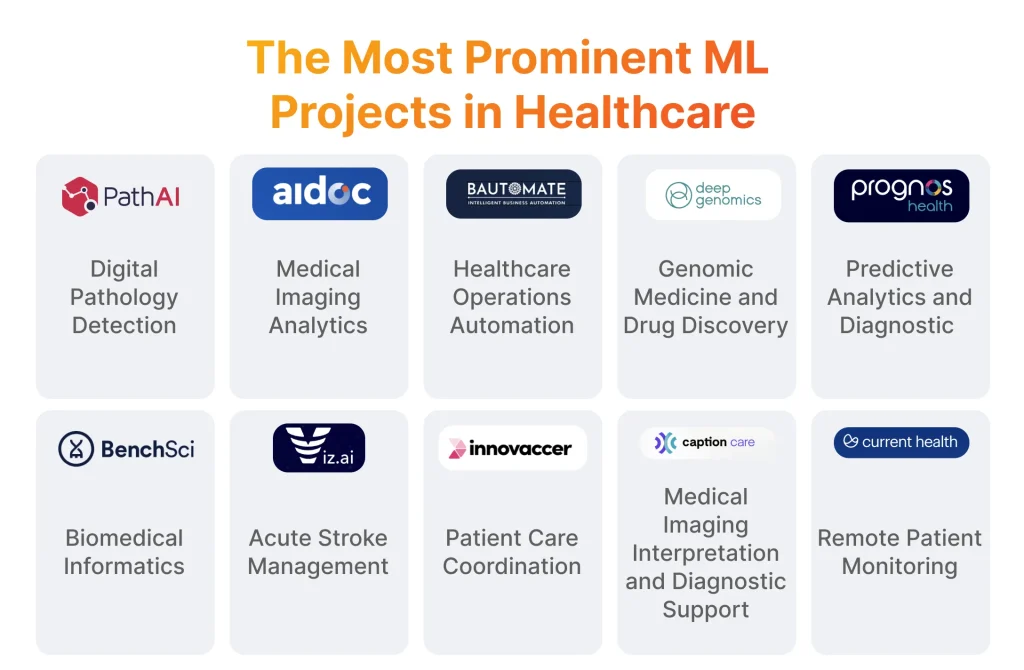
Digital Pathology Detection
PathAI offers robust AI-powered products for pathology assessment across research and clinical development. Their solutions are for the accurate diagnosis of diseases from tissue samples, including cancer research, early detection, and grading.
PathAI products include:
- PathExplore: AI-powered panel of histopathology features that spatially characterize the tumor microenvironment.
- AIM-PD-L1: Detection and quantifying PD-L1+ tumor and immune cells. Available for biopharma and lab research use.
- AIM-HER2 Breast Cancer: Automated and reproducible digital HER2 scoring with explainable AI heatmap visualizations of breast cancer cells.
- AIM-NASH: Automated, reproducible, and validated AI-powered ordinal and continuous NASH CRN score component grades and fibrosis stage.
- NASH Explore: AI-powered panel of histopathology features that spatially characterize the NASH tissue biopsy microarchitecture.
- AIM-HI Ulcerative Colitis: Automated and reproducible Geboes scoring, sub-category scoring, and conversion to Robarts and Nancy scoring for ulcerative colitis.
- IBD Explore: AI-powered panel of histopathology features that spatially characterizes the inflammatory microenvironment in ulcerative colitis.
Medical Imaging Analytics
Aidoc delivers a powerful platform for the analysis of medical images, including radiology scans, CT scans, and X-rays, to assist radiologists in identifying critical findings and abnormalities.
In addition to that, the company offers enterprise-wide AI support for organizations, which includes:
- Automated Referral Network
- Identification of Suspected Abnormalities
- Care Team Coordination
- Prioritized Discharge
- Patient Management.
Healthcare Operations Automation
Bautomate offers a business intelligence platform for Healthcare operations that aims to automate routine tasks.
In addition to the possibility of improving customer service with AI, the solution covers a wide range of operational tasks:
- Claims Processing
- Payment Processing
- Appointment Scheduling
- Compliance Management
- Medication Management
- Population Health Management
- Clinical Documentation
- Audit Procedures
- Revenue Cycle Management
- Medical Coding
Genomic Medicine and Drug Discovery Process
Deep Genomics leverages revolutions in AI, RNA biology, and automation to enable a new approach to drug discovery and development. Their AI Platform untangles the complexity of RNA biology, identifies novel targets, and evaluates thousands of possibilities to identify the best therapeutic candidates.
Predictive Analytics and Diagnostic
Prognos Health leverages AI and Big Data analysis to generate insights from clinical laboratory data, supporting early diagnosis, risk stratification, and treatment optimization. We already discussed the business impact of Big Data, so if you want to get more familiar with this concept, we suggest you read our article.
The company offers a set of solutions including:
- Prognos Oncology
- Prognos Rare Disease
- Commercial Analytics
- Precision Marketing
Biomedical Informatics
BenchSci offers the disease biology generative AI platform for pharmaceutical companies’ R&D that accelerates biomedical research and drug discovery by analyzing scientific literature, experimental data, and molecular pathways. The core of the solution is ASCEND, powered by their evidence engine, built from ontologies and multi-modal AI to understand the entire history of biomedical experiments and clinical trials with explainability and domain expertise.
Acute Stroke Management
Viz.ai offers an AI-based solution for the quick identification and triage of acute stroke cases on medical imaging, enabling timely intervention and treatment. The solution helps to significantly save time and improve patient and economic outcomes for organizations.
The solution consists of:
- Viz Neuro: for accelerated detection and treatment of suspected neurovascular diseases.
- Viz Cardio: for meeting patients’ cardiovascular needs.
- Viz Vascular: designed to transform patient outcomes into vascular medicine.
- Viz Trauma: streamlining communications and improving patient response regarding traumas.
- Viz Radiology: a suite of solutions for accelerating patient diagnosis and treatment.
Patient Care Coordination
Innovaccer offers an AI-enabled patient care coordination platform that integrates data from multiple sources, including EHRs, claims data, and wearables, to provide a comprehensive view of patient health and already helping 96,000+ medical professionals. The solutions help to unify Health Plans, DMEs, Claims, EHRs, Labs, Pharmacy, and SDoH into unified patient records.
Medical Imaging Interpretation and Diagnostic Support
Caption Care delivers AI-driven medical imaging software that enables clinicians to perform and interpret ultrasound exams more accurately and efficiently.
The solution is aimed at:
- ACOs and Value-Based Care Providers
- Health Plans
- Employer Benefit Groups
- Concierge Medicine Providers
- Gaps Closure, Home Health, and Chronic Care Solutions.
Remote Patient Monitoring
Current Health offers a remote patient monitoring platform powered by AI and predictive analytics, enabling proactive Healthcare interventions and care coordination. The platform offers Healthcare providers services like Virtual Wards, Transitional Care, and Chronic Care, while pharmaceutical companies can benefit from Decentralized Clinical Trials and Commercial Deployment.
The Challenges of Machine Learning in the Healthcare Sector
While the potential is seemingly endless, there are still some significant challenges that should be addressed while building a Healthcare system powered by Machine Learning.
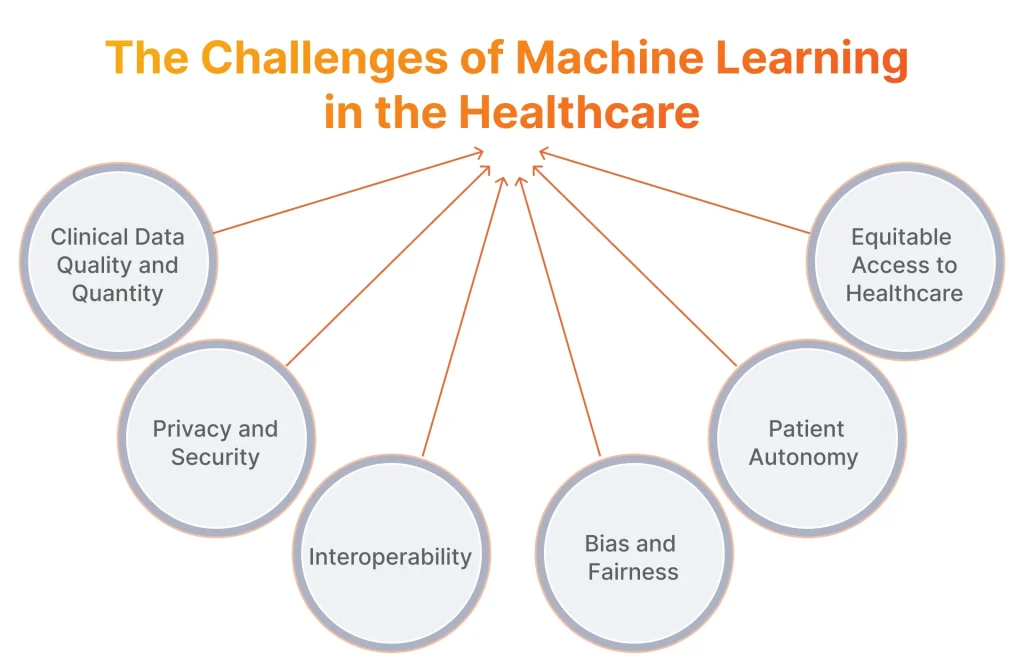
Any Machine Learning project relies heavily on accurate data, so several blockers should be discussed.
- Complexity and Unstructured Nature: Healthcare data comes in various formats including text, images, and time-series data, making it complex and unstructured. This complexity can lead to challenges in preprocessing and extracting meaningful information.
- Scattered Data Sources: Patient data is often scattered across multiple systems such as EHRs, medical devices, and administrative databases. Integrating data from these disparate sources while ensuring consistency and accuracy poses a significant challenge.
- Insufficient Quantity: Building accurate Machine Learning models requires large volumes of high-quality labeled data. However, datasets of healthcare systems are often limited in size due to privacy concerns, which we will discuss later, making it challenging to train robust models.
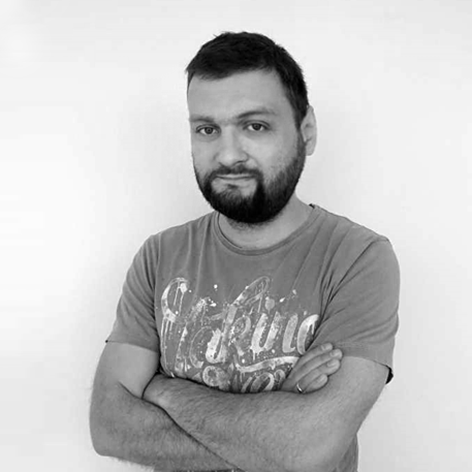
Serhii Leleko
ML & AI Engineer at SPD Technology
“There are several ways to deal with data-related challenges in your organization. First, try to implement standardized clinical data formats, coding systems, and terminologies to ensure consistency and interoperability across different systems. You can also foster collaboration among your departments to share anonymized datasets for potential training of Machine Learning models while maintaining patient privacy and compliance with regulations.”
Privacy and Security
These aspects are always of the utmost importance, especially in healthcare projects, and should be always prioritized.
- Sensitive Data: Healthcare data contains sensitive information such as patient demographics, medical history, and treatment records. Protecting this data from unauthorized access, breaches, and misuse is paramount to maintaining patient privacy and compliance with regulations.
- Regulatory Compliance: Healthcare data is subject to stringent privacy regulations such as the Health Insurance Portability and Accountability Act (HIPAA) in the US. Adhering to these regulations while utilizing data for AI applications requires implementing robust security measures, data encryption, and access controls.
Interoperability
Another important thing to consider is standardization, not only with data, as we mentioned earlier, but with all the systems involved in your organization.
- Disparate Systems and Standards: Healthcare organizations often use different systems and protocols. Integrating Machine Learning systems with existing infrastructure and achieving interoperability between disparate systems could be challenging if you don’t make an effort to prepare for this.
- Data Silos: Existing data silos within your organization contain a lot of information that could be used for Machine Learning solutions. Breaking down these silos and establishing interoperable frameworks is essential for leveraging AI to its fullest potential.
Ethical Issues and Concerns of Using ML and AI in Medical Services and Research
From an ethical standpoint, there is a lot to talk about. Some of the issues we are going to discuss are holding the evolution of Machine Learning in Healthcare back to some degree.
Bias and Fairness
Healthcare data often reflects historical disparities and inequalities, leading to biased representations of information on certain patients. For example, racial or socioeconomic biases may be present in diagnostic patient records or treatment decisions. AI models trained on biased data can amplify these disparities, leading to unequal healthcare outcomes for different groups. For instance, an AI algorithm for predicting disease risk may disproportionately misclassify certain demographic groups.
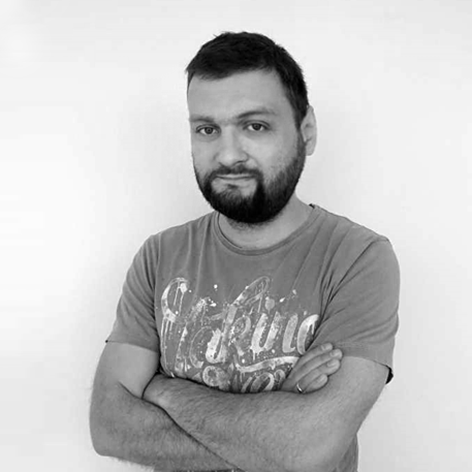
Serhii Leleko
ML & AI Engineer at SPD Technology
“To address bias in AI algorithms, it’s essential to carefully evaluate training data for biases, implement bias detection techniques during model development, and employ fairness-aware algorithms that explicitly account for fairness constraints during training.”
Patient Autonomy and Informed Consent
Patients may be unaware of how their health data is being used to train AI models or influence clinical decision-making. This lack of transparency can undermine patient autonomy and decision-making agency. Obtaining informed consent from patients for the use of their data in AI applications, however, is quite challenging due to the complexity of AI algorithms and the technical nature of Machine Learning processes.
To deal with it, Healthcare professionals should prioritize patient education and communication to ensure that patients understand the implications of AI in healthcare, including how their data is used and the potential impact on their care. Ensuring transparency and explainability in AI models is essential for building trust among patients, healthcare professionals, and regulators.
Equitable Access and Resource Allocation
The implementation of Machine Learning technology may widen existing disparities in access to healthcare services and resources, particularly in underserved communities with limited access to technology. AI-driven Healthcare interventions may disproportionately benefit well-resourced Healthcare institutions.
To promote equitable access to AI-driven healthcare solutions, it’s essential to engage with local communities, prioritize community needs and preferences, and develop culturally sensitive and contextually appropriate interventions.
Machine Learning is a trending technology in other industries as well.
To discover how it impacts other niches, consider our guide on Artificial Intelligence and Machine Learning in Manufacturing.
The Future Applications of AI-enabled Technology in Healthcare
The Global Healthcare IT market is expected to reach USD 974.5 billion by 2027, and AI technology is a major part of this growth. In this section, we will talk about the applications that have the most potential to explode soon.
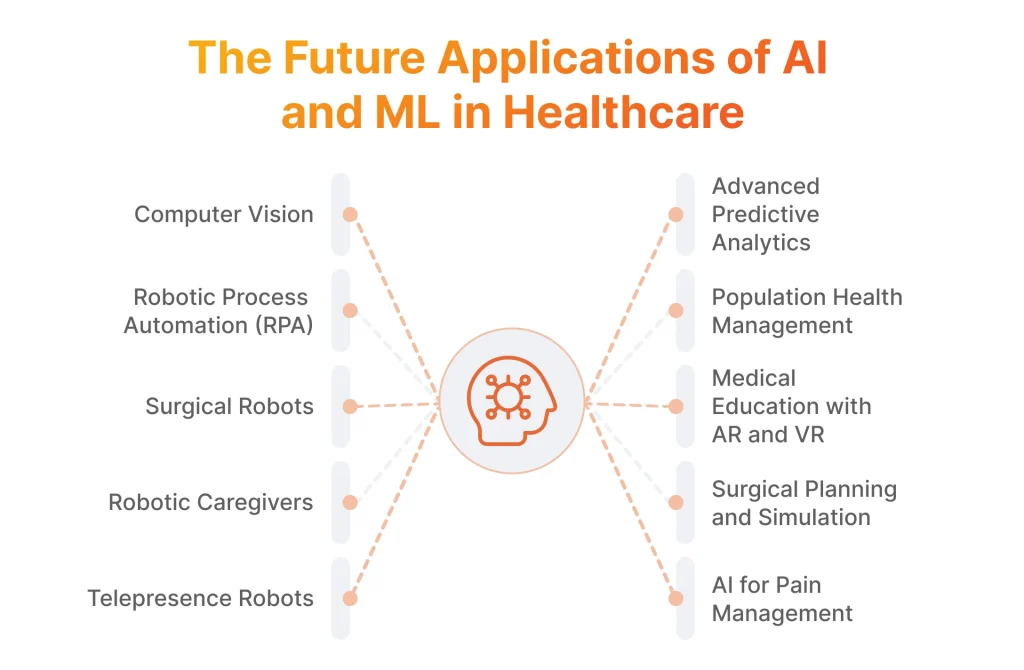
Computer Vision
A branch of AI that enables machines to interpret and analyze visual information from the real world, is increasingly being applied in various areas within the healthcare industry including:
- Analyze Medical Images
- Surgical Assistance and Navigation
- Health Monitoring and Remote Patient Care
- Drug Development, Discovery, and Pharmacology
As a part of one of our recent projects, we successfully created a computer vision-powered API for a petcare mobile app. Our team successfully addressed noisy labeled data and its high imbalance between classes, ensuring data consistency and reliability for subsequent neural network development to achieve the goal of the project.
Robotic Process Automation (RPA)
AI-powered robots can automate repetitive administrative tasks such as appointment scheduling, insurance claims processing, and EHR management. By reducing manual intervention and streamlining administrative workflows, RPA increases operational efficiency and reduces the administrative burden on healthcare staff.
In addition to that, robots can perform tasks with a high degree of accuracy and consistency, minimizing errors and improving data quality in administrative processes, so there is no surprise that the Global RPA Market is projected to reach USD 13.39 billion by 2030.
Surgical Robots
Advanced robotic systems assist surgeons in performing minimally invasive surgeries with greater precision and dexterity, reducing surgical trauma and improving patient health outcomes. Robotic surgery provides enhanced visualization capabilities, allowing surgeons to operate with greater precision and control, especially in complex procedures.
Robotic Caregivers
Robots equipped with AI capabilities provide personalized patient care, assisting with activities of daily living, medication management, and rehabilitation exercises. Robotic caregivers offer round-the-clock support and monitoring, ensuring continuous care for patients in healthcare facilities or at home. Some robotic caregivers even serve as companions for patients, offering social interaction and emotional support, which is especially important for elderly or isolated individuals.
Telepresence Robots
Remote-controlled robots enable healthcare providers to conduct virtual consultations, improving access to care for patients in remote or underserved areas. Telepresence robots facilitate medical education and training by enabling remote observation and participation in clinical trials, procedures, and consultations.
Thanks to this innovation, patients can interact with healthcare professionals and receive personalized care remotely, enhancing patient engagement and adherence to treatment plans.
Another industry where AI-driven remote-controlled robots can be very useful is the Food industry. Read our guide on Machine Learning and AI in the Food Industry for more advanced applications of this technology.
Predictive Analytics and Population Health Management
Just like successful implementations in other industries, for example, AI for customer behavior analysis in Retail, Machine Learning techniques in healthcare for predictive analytics are quite powerful. Combined with blockchain-enabled data sharing, predictive analytics help with disease outbreaks and the identification of at-risk populations, improving public health surveillance. Predictive analytics optimize resource allocation by forecasting healthcare demands, identifying areas of need, and allocating resources efficiently to improve population health outcomes.
Medical Training and Education with AR and VR
AR and VR Healthcare technologies simulate realistic medical scenarios, allowing healthcare professionals to practice skills and procedures in a safe and controlled environment. Medical training programs utilizing AR and VR simulations are a great way to enhance skill development, decision-making, and teamwork.
Surgical Planning and Simulation
With the help of AR and VR technologies, surgeons can leverage immersive 3D visualization of patient anatomy, enabling better surgical planning and decision-making. Surgeons implement complex procedures in their medical practice in virtual environments, refining their techniques and optimizing surgical plans before performing them in the operating room. Surgical planning and simulation ultimately reduce the risk of intraoperative complications, leading to improved patient outcomes, shorter recovery times, and reduced healthcare costs.
Pain Management and Therapy
Finally, VR environments can distract patients from pain during medical procedures, reducing the need for sedation and opioid medications. VR-based rehabilitation programs can support patients with chronic pain or physical disabilities by providing immersive therapy experiences and personalized treatment plans.
Conclusion
The integration of Machine Learning in healthcare represents a groundbreaking paradigm shift, already bringing transformative benefits across the entire industry. By harnessing the power of advanced algorithms and vast datasets, Machine Learning holds the potential to continue revolutionizing medical diagnostics, treatment planning, patient management, and medical research.
We can help you navigate the complexities of this growing technological innovation, and leverage its power in your medical organization. If you have any questions or need consultation, our tech and business-savvy specialists would be glad to help you!
FAQ
- What is Machine Learning in healthcare?
It is a usage of computational algorithms and statistical models to analyze and interpret complex medical historical data, extract meaningful insights, make predictions, and aid clinical decision-making processes. It leverages large datasets, including Electronic Health Records (EHRs), medical images, genetic information, patient-reported data, and more, to improve patient outcomes, optimize healthcare delivery, and advance medical research to deliver results beyond human capabilities.
- How is Machine Learning used in healthcare?
It can be used in various ways, and as some new implementations emerge, we can highlight the following use cases of this innovation:
- Medical Imaging Analysis
- Deep Learning for Predictive Analytics and Risk Stratification
- Personalized Medicine
- Clinical Decision Support Systems
- Healthcare Operations and Resource Management
- Drug Discovery and Development
- Robotic Surgery.
- Why is Machine Learning important in healthcare?
Machine Learning is instrumental in transforming the healthcare sector by leveraging data-driven insights. It leads to enhancing patient outcomes, optimizing resource utilization, accelerating medical research, and empowering healthcare professionals with advanced decision-support tools. Such ML advancements as deep Learning, Natural Language Processing, and Big Data are expected to deliver even more value in the near future.